Visual similarity learning method based on depth unsupervised learning
A technology of unsupervised learning and learning methods, applied in the fields of instruments, character and pattern recognition, computer parts, etc., can solve the problem of high computational cost
- Summary
- Abstract
- Description
- Claims
- Application Information
AI Technical Summary
Problems solved by technology
Method used
Image
Examples
Embodiment Construction
[0029] It should be noted that, in the case of no conflict, the embodiments in the present application and the features in the embodiments can be combined with each other. The present invention will be further described in detail below in conjunction with the drawings and specific embodiments.
[0030] figure 1 It is a system flowchart of a visual similarity learning method based on deep unsupervised learning in the present invention. It mainly includes the generation of compact clusters and batches, the training of convolutional neural networks (CNN), local temporal pooling and multi-instance learning.
[0031] Among them, the generation of compact clusters and batches means that after obtaining the associated initial sample set from the samples, by optimizing a single cost function, a compact cluster (sample group with compact and similar position distribution) is obtained, and then the selection of similarity mutual Consistent clusters make up a stochastic gradient descent...
PUM
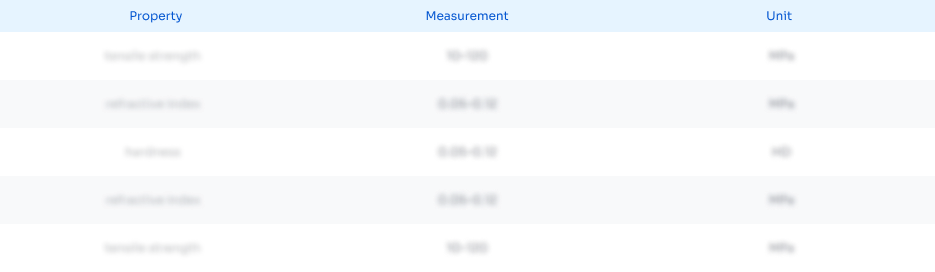
Abstract
Description
Claims
Application Information
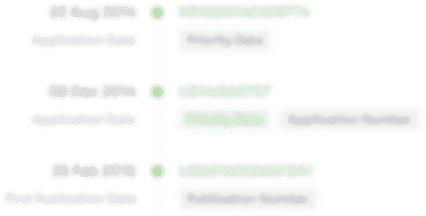
- R&D
- Intellectual Property
- Life Sciences
- Materials
- Tech Scout
- Unparalleled Data Quality
- Higher Quality Content
- 60% Fewer Hallucinations
Browse by: Latest US Patents, China's latest patents, Technical Efficacy Thesaurus, Application Domain, Technology Topic, Popular Technical Reports.
© 2025 PatSnap. All rights reserved.Legal|Privacy policy|Modern Slavery Act Transparency Statement|Sitemap|About US| Contact US: help@patsnap.com