A sequential modeling method based on split-loop neural network
A cyclic neural network and model building technology, applied in biological neural network models, neural learning methods, neural architectures, etc., can solve problems such as slow RNN speed, improve accuracy, improve training speed, and improve the ability to retain important information Effect
- Summary
- Abstract
- Description
- Claims
- Application Information
AI Technical Summary
Problems solved by technology
Method used
Image
Examples
Embodiment Construction
[0061] The present invention will be described in detail below in conjunction with specific embodiments. The following examples will help those skilled in the art to further understand the present invention, but do not limit the present invention in any form. It should be noted that those skilled in the art can make several changes and improvements without departing from the concept of the present invention. These all belong to the protection scope of the present invention.
[0062] The present invention defines the following technical terms: RNN (Recurrent neural network): cyclic neural network; CNN (Convolutional neural network): convolutional neural network; LSTM (Long-short term memory): long-term short-term memory network; GRU (Gated recurrent unit ): Gated recurrent unit; SRNN (Sliced recurrent neural network): Sliced recurrent neural network.
[0063] Figure 4 It is a schematic diagram of the structure of SRNN, and the present invention is as follows Figure 4 ...
PUM
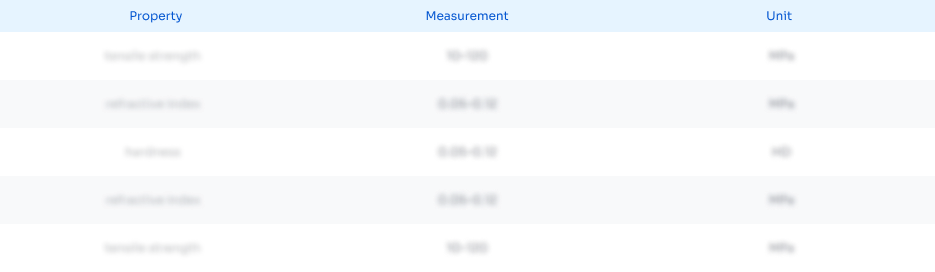
Abstract
Description
Claims
Application Information
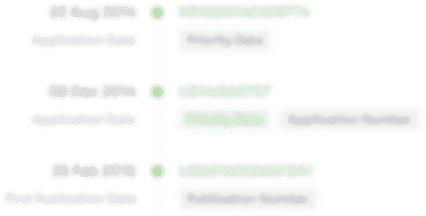
- R&D
- Intellectual Property
- Life Sciences
- Materials
- Tech Scout
- Unparalleled Data Quality
- Higher Quality Content
- 60% Fewer Hallucinations
Browse by: Latest US Patents, China's latest patents, Technical Efficacy Thesaurus, Application Domain, Technology Topic, Popular Technical Reports.
© 2025 PatSnap. All rights reserved.Legal|Privacy policy|Modern Slavery Act Transparency Statement|Sitemap|About US| Contact US: help@patsnap.com