Brain tumor segmentation algorithm based on UNet++ optimization and weight budget
A segmentation algorithm, brain tumor technology, applied in the field of brain tumor segmentation algorithm, can solve the problems of low segmentation accuracy, incomplete semantic information of UNet network, slow training speed, etc., achieve good segmentation effect and solve the effect of slow network training speed
- Summary
- Abstract
- Description
- Claims
- Application Information
AI Technical Summary
Problems solved by technology
Method used
Image
Examples
Embodiment Construction
[0060] For those skilled in the art, it is understandable that some well-known structures and descriptions thereof may be omitted in the drawings. The technical solutions of the present invention will be further described below in conjunction with the accompanying drawings and embodiments.
[0061] The present invention provides a brain tumor segmentation algorithm based on UNet++ optimization and weight budgeting. The method realizes the segmentation of the whole tumor, tumor core and enhanced tumor core of brain tumors, and provides high-precision segmentation of brain tumor MRI images. Repeatable measurements and evaluations provide more accurate tumor image segmentation maps.
[0062] figure 1 It is the flow chart of the method of the present invention, first image preprocessing, BraTS2018 and BraTS2019 become the input required by the network; then build an improved UNet network model, and use it to train the data, and save the network weight with the best effect; Next,...
PUM
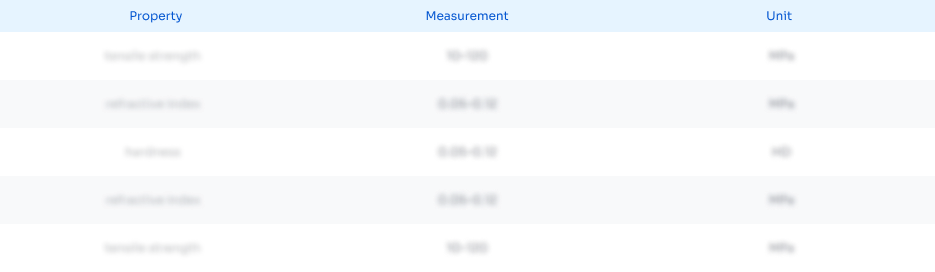
Abstract
Description
Claims
Application Information
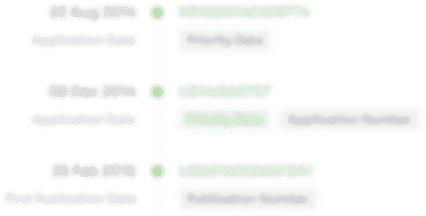
- R&D Engineer
- R&D Manager
- IP Professional
- Industry Leading Data Capabilities
- Powerful AI technology
- Patent DNA Extraction
Browse by: Latest US Patents, China's latest patents, Technical Efficacy Thesaurus, Application Domain, Technology Topic, Popular Technical Reports.
© 2024 PatSnap. All rights reserved.Legal|Privacy policy|Modern Slavery Act Transparency Statement|Sitemap|About US| Contact US: help@patsnap.com