Image Semantic Segmentation Method Based on Global Features and Local Features of Deep Learning
A technology of global features and local features, applied in the field of semantic segmentation of computer vision images, can solve problems such as the difficulty of widely representing image features, achieve the effects of reducing noise, clear boundaries, and improving accuracy
- Summary
- Abstract
- Description
- Claims
- Application Information
AI Technical Summary
Problems solved by technology
Method used
Image
Examples
Embodiment Construction
[0034] Embodiments of the present invention will be described in further detail below in conjunction with the accompanying drawings.
[0035] An image semantic segmentation method based on global features and local features of deep learning, such as Figure 1 to Figure 3 As shown, at the encoding end, the deep convolutional neural network model is used to extract the global and local features of the image; at the decoding end, the two features are fused to obtain complementary image discriminant features for image semantic segmentation. At the same time, in order to restore the original resolution of the image more accurately at the decoding end, a stacked pooling layer is proposed. The image features are stacked through the maximum pooling layer, the convolutional layer, and finally the anti-pooling layer. The feature is fused with the previous convolution feature to reduce the noise in the feature map, make the boundary of the segmentation map clearer, and also reduce the cl...
PUM
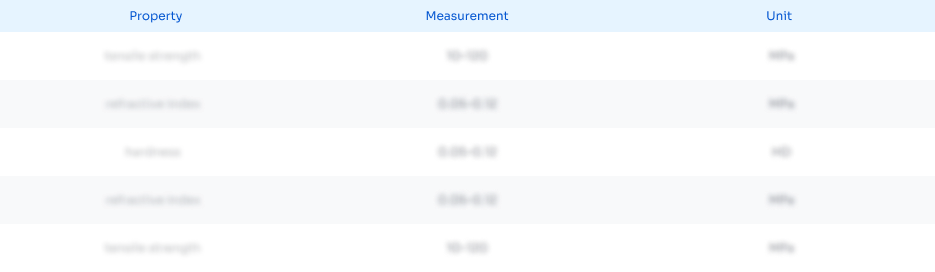
Abstract
Description
Claims
Application Information
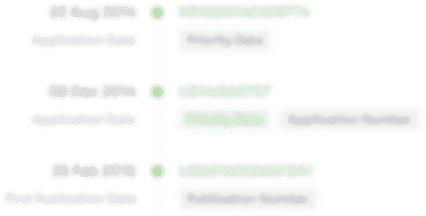
- R&D Engineer
- R&D Manager
- IP Professional
- Industry Leading Data Capabilities
- Powerful AI technology
- Patent DNA Extraction
Browse by: Latest US Patents, China's latest patents, Technical Efficacy Thesaurus, Application Domain, Technology Topic, Popular Technical Reports.
© 2024 PatSnap. All rights reserved.Legal|Privacy policy|Modern Slavery Act Transparency Statement|Sitemap|About US| Contact US: help@patsnap.com