Hybrid depth learning model LSTM-ResNet based metropolitan space-time flow prediction technology
A technology of deep learning and prediction technology, applied in the field of geographic information, can solve problems affecting the accuracy of spatio-temporal feature capture and ignore the dependencies of spatio-temporal units before and after, so as to achieve good prediction results, accurate capture, and improve the effect of capture accuracy
- Summary
- Abstract
- Description
- Claims
- Application Information
AI Technical Summary
Problems solved by technology
Method used
Image
Examples
Embodiment Construction
[0019] The metropolitan space-time flow prediction technology based on the hybrid deep learning model LSTM-ResNet of the present invention (such as figure 1 shown), including the following steps:
[0020] (1) Process the input dataset into three forms: a spatiotemporal flow series with intervals of hourly, daily and weekly patterns. Assume that the time interval of the predicted target is t th , the total number of time intervals in one day is m, the radius of the time buffer is b, and the space-time flow data of the i-th time interval is a three-dimensional tensor X i . Input data , and They are:
[0021]
[0022]
[0023]
[0024] (2) The LSTM model filters out invalid temporal features from the input spatiotemporal flow sequence. Feed the original space-time flow sequence into the multi-layer LSTM model, through Transform the data and finally get the candidate feature map (O represents the number of neurons in the LSTM layer, and M×N represents the gr...
PUM
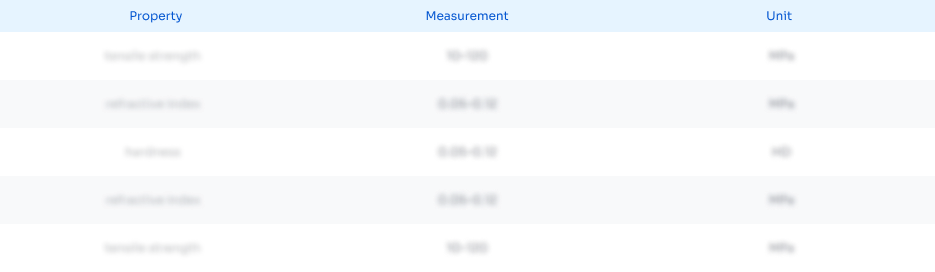
Abstract
Description
Claims
Application Information
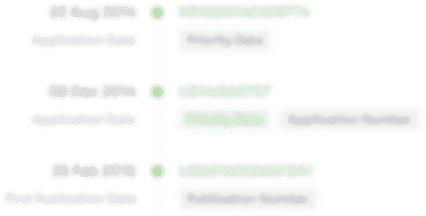
- R&D
- Intellectual Property
- Life Sciences
- Materials
- Tech Scout
- Unparalleled Data Quality
- Higher Quality Content
- 60% Fewer Hallucinations
Browse by: Latest US Patents, China's latest patents, Technical Efficacy Thesaurus, Application Domain, Technology Topic, Popular Technical Reports.
© 2025 PatSnap. All rights reserved.Legal|Privacy policy|Modern Slavery Act Transparency Statement|Sitemap|About US| Contact US: help@patsnap.com