Method, device, computing device, and computer storage medium for allocating display memory
A device and data storage technology, applied in the computer field, can solve problems such as low utilization rate, high implementation cost, and the inability of the graphics card to load multiple deep learning algorithm models, so as to achieve the effect of reducing implementation cost and video memory resources
- Summary
- Abstract
- Description
- Claims
- Application Information
AI Technical Summary
Problems solved by technology
Method used
Image
Examples
Embodiment Construction
[0026] Exemplary embodiments of the present disclosure will be described in more detail below with reference to the accompanying drawings. Although exemplary embodiments of the present disclosure are shown in the drawings, it should be understood that the present disclosure may be embodied in various forms and should not be limited by the embodiments set forth herein. Rather, these embodiments are provided for more thorough understanding of the present disclosure and to fully convey the scope of the present disclosure to those skilled in the art.
[0027] The deep learning algorithm model is a highly modular and modular algorithm model. Currently commonly used deep learning frameworks such as Caffe, Mxnet, tensorflow, etc., their design concept is to abstract the deep learning algorithm model into a calculation roadmap ( It is a defined directed acyclic graph), and then control the input data flow of the algorithm model, and calculate layer by layer, and each layer will have a...
PUM
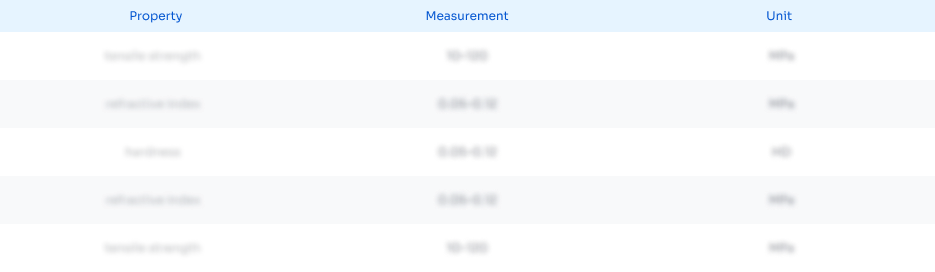
Abstract
Description
Claims
Application Information
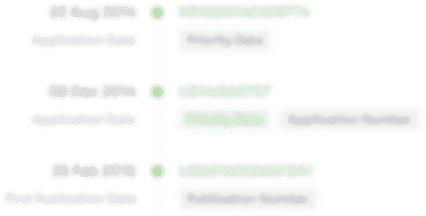
- R&D Engineer
- R&D Manager
- IP Professional
- Industry Leading Data Capabilities
- Powerful AI technology
- Patent DNA Extraction
Browse by: Latest US Patents, China's latest patents, Technical Efficacy Thesaurus, Application Domain, Technology Topic.
© 2024 PatSnap. All rights reserved.Legal|Privacy policy|Modern Slavery Act Transparency Statement|Sitemap