A cell defect classification method based on unbiased embedding zero-sample learning
A defect classification and sample learning technology, applied in image analysis, computer parts, image data processing, etc., can solve problems such as strong bias, insufficient defect classifier training, and small sample size, and achieve the effect of ensuring classification accuracy.
- Summary
- Abstract
- Description
- Claims
- Application Information
AI Technical Summary
Problems solved by technology
Method used
Image
Examples
Embodiment Construction
[0024] The technical solution of the present invention is clearly and completely explained and described below.
[0025] The present invention proposes an unbiased embedded zero-sample learning battery defect classification method, which can realize the classification of common defects (source category) and rare defects (target category) for image blocks containing battery defects, including the following steps :
[0026] Step 1, collecting image data of cell defects. The battery cell is photographed by a high-definition camera, and the center point is randomly searched for the part containing defects, and cut into 96×96 image blocks, while ensuring that various defects can still be seen in the image blocks. Mark the cut image blocks as pits, damaged single-layer isolation film, folded isolation film, scratches, folded tabs, glue stains, damaged metal leaks, air bubbles, toner strips, toner dirt, crushing, There are 13 types of cell defect categories including foreign matter...
PUM
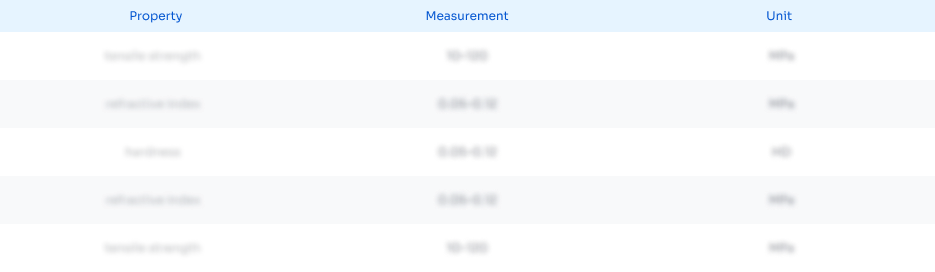
Abstract
Description
Claims
Application Information
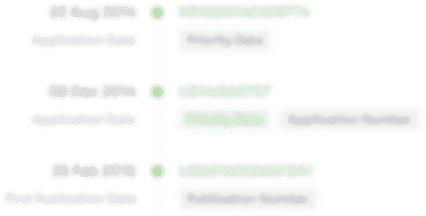
- R&D Engineer
- R&D Manager
- IP Professional
- Industry Leading Data Capabilities
- Powerful AI technology
- Patent DNA Extraction
Browse by: Latest US Patents, China's latest patents, Technical Efficacy Thesaurus, Application Domain, Technology Topic, Popular Technical Reports.
© 2024 PatSnap. All rights reserved.Legal|Privacy policy|Modern Slavery Act Transparency Statement|Sitemap|About US| Contact US: help@patsnap.com