Remote sensing image classification network robustness improvement method based on self-supervised learning
A remote sensing image and classification network technology, applied in the intersection of deep learning and remote sensing, can solve problems such as improving model performance with remote sensing resources, and achieve the effects of defense, performance improvement, and robustness enhancement.
- Summary
- Abstract
- Description
- Claims
- Application Information
AI Technical Summary
Problems solved by technology
Method used
Image
Examples
Embodiment 1
[0052] At present, deep learning technology has made great progress and has been deeply integrated with remote sensing technology to achieve revolutionary results. Hidden danger. The adversarial attack can make the output of the deep network completely different from the original result by adding carefully designed tiny noise to the original image, which seriously threatens the security of remote sensing detection and recognition.
[0053] In remote sensing detection and recognition, it is necessary to face a large number of natural noises, such as cloud and fog occlusion, focus blur, wind, frost, rain and snow, and digital noise, as well as well-designed artificial interference such as military concealment, which puts forward the robustness of the model. Very demanding.
[0054] In this regard, this embodiment provides a method for improving the robustness of a remote sensing image classification network based on self-supervised learning, such as figure 1 and figure 2 As ...
PUM
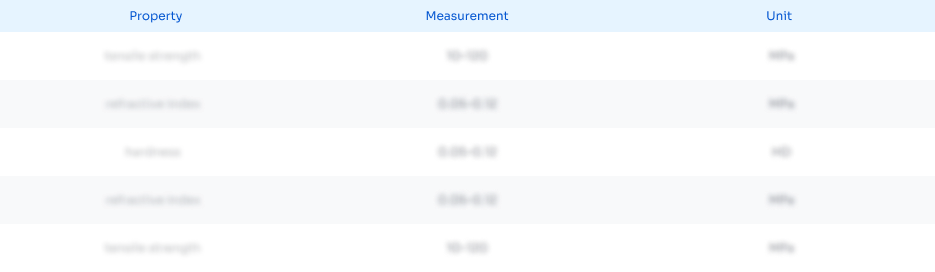
Abstract
Description
Claims
Application Information
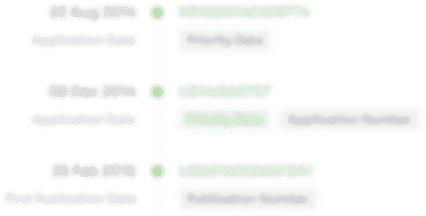
- R&D
- Intellectual Property
- Life Sciences
- Materials
- Tech Scout
- Unparalleled Data Quality
- Higher Quality Content
- 60% Fewer Hallucinations
Browse by: Latest US Patents, China's latest patents, Technical Efficacy Thesaurus, Application Domain, Technology Topic, Popular Technical Reports.
© 2025 PatSnap. All rights reserved.Legal|Privacy policy|Modern Slavery Act Transparency Statement|Sitemap|About US| Contact US: help@patsnap.com