Remote sensing image classification method based on partially random supervision discrete Hash
A technology of remote sensing images and classification methods, which is applied in computer parts, character and pattern recognition, instruments, etc., can solve the problems of large amount of remote sensing image data and complicated calculation, and achieves reduction of computational complexity, guaranteed classification accuracy, and effective The effect of using
- Summary
- Abstract
- Description
- Claims
- Application Information
AI Technical Summary
Problems solved by technology
Method used
Image
Examples
Embodiment Construction
[0021] The present invention combines two major categories of methods in hash learning—data-independent methods and data-dependent methods. The method combines a generative model of discrete binary codes with a partially stochastic constrained model. Through random projection, the problem of high computational complexity caused by the large amount of remote sensing image data can be solved, and through the weight matrix generated by training data, the semantics between data can be well preserved in the generation process of hash coding similarity. For the optimization problem of the objective function, this method adopts the cyclic iterative optimization method to iteratively optimize the parameters, and decomposes the optimization process into three steps, so as to solve the problem of multi-variable optimal solution. In the hash code generation process, this method adopts the discrete cyclic coordinate descent method, in this way, the code can be optimized bit by bit, so as...
PUM
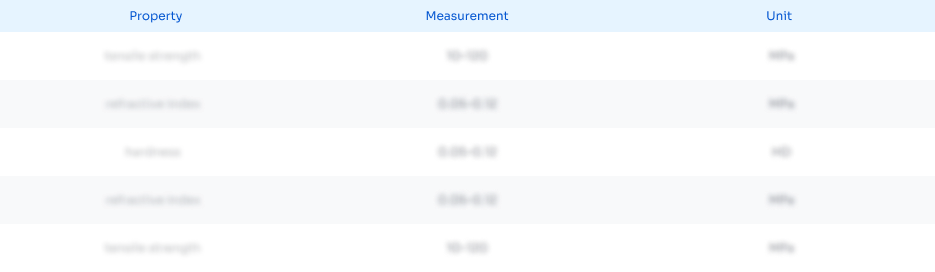
Abstract
Description
Claims
Application Information
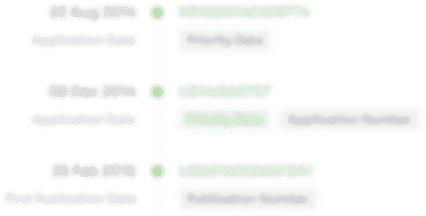
- Generate Ideas
- Intellectual Property
- Life Sciences
- Materials
- Tech Scout
- Unparalleled Data Quality
- Higher Quality Content
- 60% Fewer Hallucinations
Browse by: Latest US Patents, China's latest patents, Technical Efficacy Thesaurus, Application Domain, Technology Topic, Popular Technical Reports.
© 2025 PatSnap. All rights reserved.Legal|Privacy policy|Modern Slavery Act Transparency Statement|Sitemap|About US| Contact US: help@patsnap.com