A double-clustering recommendation method and system based on reinforcement learning
A recommendation method and reinforcement learning technology, applied in the field of recommendation, can solve problems such as unsatisfactory recommendation effect and poor user experience
- Summary
- Abstract
- Description
- Claims
- Application Information
AI Technical Summary
Problems solved by technology
Method used
Image
Examples
Embodiment 1
[0046] In this example, if figure 1 As shown, a biclustering recommendation method based on reinforcement learning includes the following steps:
[0047] S1. Establish a user-item matrix;
[0048] Use the behavior of the user to click on the recommended items (filters, stickers) to construct the user-item matrix. Assume that the user has N=5 and the items M=12, and construct an N×M user-item matrix, as shown in Table 1 .
[0049] Table 1:
[0050] Item 1 Item 2 Item 3 Item 4 item 5 Item 6 Item 7 Item 8 Item 9 item 10 Item 11 Item 12 user 1 1 0 0 1 0 1 1 0 1 1 1 0 user 2 1 1 1 1 0 0 0 1 0 1 1 0 user 3 1 1 1 1 1 1 1 0 0 1 1 0 user 4 1 1 1 1 0 0 0 1 0 1 1 0 user 5 1 1 0 0 0 1 1 1 0 1 1 0
[0051] S2. Performing biclustering on the user-item matrix to obtain a plurality of biclustering clusters;
[0052] Using the BiBit double clustering method, first enco...
Embodiment 2
[0105] A biclustering recommendation system based on reinforcement learning, including:
[0106] A double-clustering module, configured to double-cluster the user-item matrix to obtain a double-cluster cluster;
[0107] A state mapping module, configured to map the biclusters to a low-dimensional space to obtain a state set;
[0108] The reinforcement learning module is used to obtain the best state transition sequence according to the state collection through reinforcement learning;
[0109] The recommendation module is used to generate a recommendation list of users to be recommended.
PUM
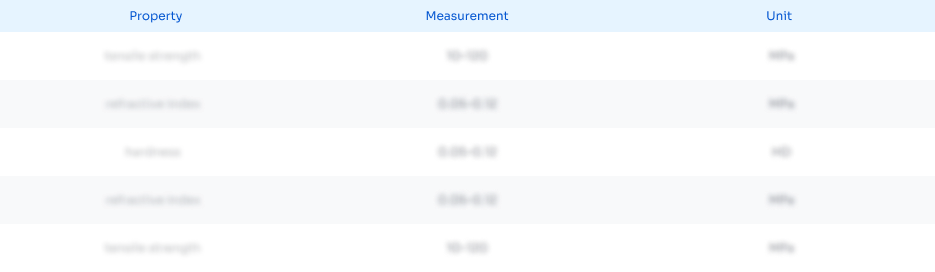
Abstract
Description
Claims
Application Information
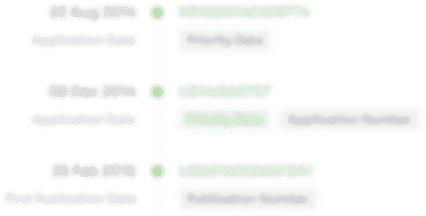
- R&D Engineer
- R&D Manager
- IP Professional
- Industry Leading Data Capabilities
- Powerful AI technology
- Patent DNA Extraction
Browse by: Latest US Patents, China's latest patents, Technical Efficacy Thesaurus, Application Domain, Technology Topic.
© 2024 PatSnap. All rights reserved.Legal|Privacy policy|Modern Slavery Act Transparency Statement|Sitemap