Semantic segmentation network integrating multi-scale feature space and semantic space
A multi-scale feature and semantic segmentation technology, applied in the field of scene understanding, can solve the problem of not considering the structural differences of different types of adjacent pixel areas without considering the structure of the same continuous pixel area, and achieve the effect of high resolution
- Summary
- Abstract
- Description
- Claims
- Application Information
AI Technical Summary
Problems solved by technology
Method used
Image
Examples
Embodiment Construction
[0021] In order to improve the semantic segmentation performance of small objects, object details and pixels near the edge, the present invention proposes a semantic segmentation network that integrates multi-scale feature space and semantic space, and realizes an end-to-end high-performance semantic segmentation system based on this network. The network adopts the form of full convolution, which allows the input image to be of any scale. It only needs to be properly supplemented at the edge so that the length and width of the image can be divisible by the maximum downsampling multiple of the network. Among them, the multi-scale feature space refers to the multi-scale feature map generated by the network feature extraction part through multi-layer convolution and downsampling, and the multi-scale semantic space refers to the prediction map obtained by supervising multiple scales of the network. The main structure of the network is as figure 2shown. Our proposed network is ma...
PUM
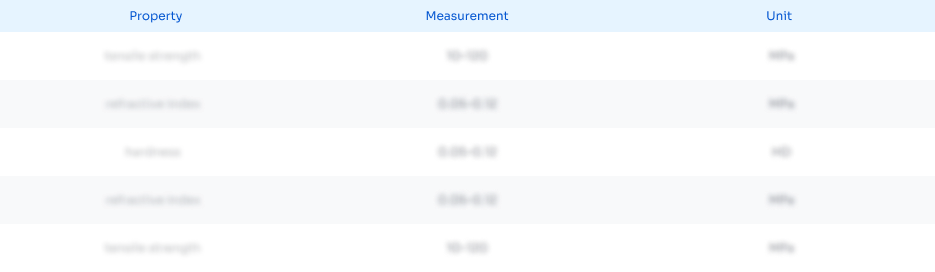
Abstract
Description
Claims
Application Information
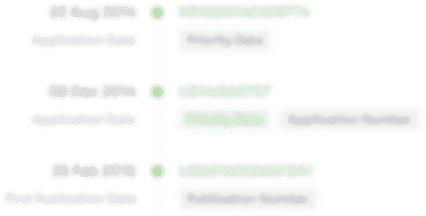
- Generate Ideas
- Intellectual Property
- Life Sciences
- Materials
- Tech Scout
- Unparalleled Data Quality
- Higher Quality Content
- 60% Fewer Hallucinations
Browse by: Latest US Patents, China's latest patents, Technical Efficacy Thesaurus, Application Domain, Technology Topic, Popular Technical Reports.
© 2025 PatSnap. All rights reserved.Legal|Privacy policy|Modern Slavery Act Transparency Statement|Sitemap|About US| Contact US: help@patsnap.com