A semantic image restoration method based on a DenseNet generative adversarial network
A repair method and image generation technology, applied in the field of deep learning and image processing, can solve problems such as the accuracy of the repair area and the visual effect that need to be improved
- Summary
- Abstract
- Description
- Claims
- Application Information
AI Technical Summary
Problems solved by technology
Method used
Image
Examples
Embodiment 1
[0074] Method of the present invention comprises the following steps:
[0075] Step 1. Data preprocessing stage. Set the size of the collected data to obtain the size required for training.
[0076] Step 2. Build the stage of generating confrontation network. The generation confrontation network model is composed of a generation network G and a discriminative network D. The generation network G adopts a network structure in which the encoder is connected to the decoder, where the encoder is composed of a DenseNet module and a convolutional layer, and the decoder is composed of a DenseNet module and a deconvolution layer. At the same time, there is a skip-connection between the encoder and the decoder. The encoder down-samples the input image to extract abstract features. The decoder upsamples the encoded feature data through deconvolution to make the output data size consistent with the input image size. The discriminant network is composed of convolutional layer and full...
PUM
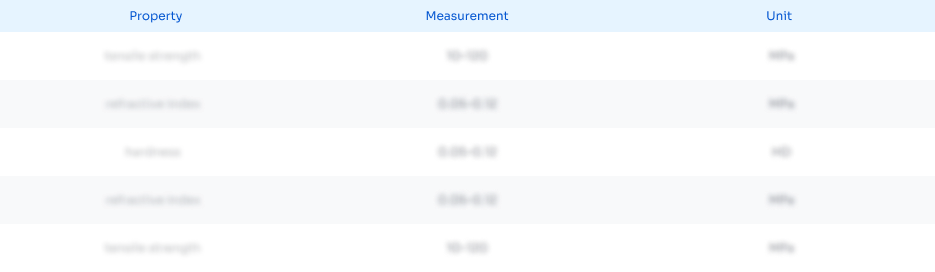
Abstract
Description
Claims
Application Information
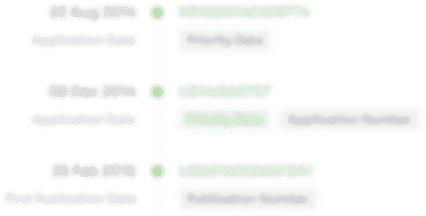
- R&D Engineer
- R&D Manager
- IP Professional
- Industry Leading Data Capabilities
- Powerful AI technology
- Patent DNA Extraction
Browse by: Latest US Patents, China's latest patents, Technical Efficacy Thesaurus, Application Domain, Technology Topic, Popular Technical Reports.
© 2024 PatSnap. All rights reserved.Legal|Privacy policy|Modern Slavery Act Transparency Statement|Sitemap|About US| Contact US: help@patsnap.com