Deep model training method and device, electronic equipment and storage medium
A model training and model technology, applied in the information field, can solve problems such as manual labeling of labeling data, model classification or recognition ability accuracy is not as expected, and labeling accuracy is difficult to guarantee, so as to achieve high classification or recognition accuracy and reduce learning abnormalities Phenomenon, the effect of fast model training
- Summary
- Abstract
- Description
- Claims
- Application Information
AI Technical Summary
Problems solved by technology
Method used
Image
Examples
example 1
[0122] Mutual learning weak supervision algorithm, using the surrounding rectangular frame of some objects in the picture as input, the two models learn from each other, and can output the pixel segmentation results of the object in other unknown pictures.
[0123] Taking cell segmentation as an example, at the beginning, some cells in the figure are marked with enclosing rectangles. Observation found that most of the cells are ellipses, so draw the largest inscribed ellipse in the rectangle, draw dividing lines between different ellipses, and draw dividing lines on the edges of the ellipses. as an initial supervisory signal. Train two segmentation models. Then the segmentation model predicts on this image, and the obtained prediction image and the initial label image are combined as a new supervisory signal. The two models use the integration results of each other, and then repeat the training of the segmentation model, so the segmentation in the image is found The results ...
PUM
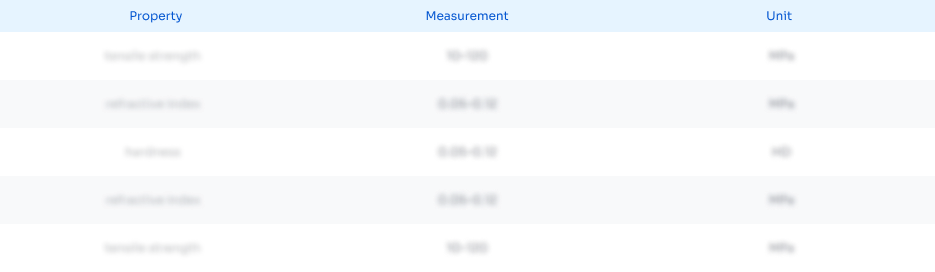
Abstract
Description
Claims
Application Information
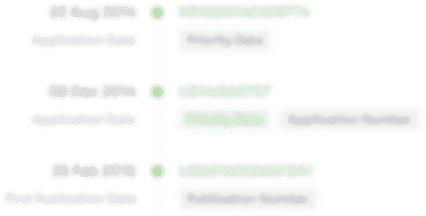
- R&D Engineer
- R&D Manager
- IP Professional
- Industry Leading Data Capabilities
- Powerful AI technology
- Patent DNA Extraction
Browse by: Latest US Patents, China's latest patents, Technical Efficacy Thesaurus, Application Domain, Technology Topic, Popular Technical Reports.
© 2024 PatSnap. All rights reserved.Legal|Privacy policy|Modern Slavery Act Transparency Statement|Sitemap|About US| Contact US: help@patsnap.com