A crowd counting method based on deep learning target detection
A target detection and deep learning technology, applied in the field of crowd counting based on deep learning target detection, can solve the problems of reduced performance and accuracy, less applicable, redundant, etc., to achieve accurate detection of the number of people, improved detection speed, and improved accuracy. degree of effect
- Summary
- Abstract
- Description
- Claims
- Application Information
AI Technical Summary
Problems solved by technology
Method used
Image
Examples
Embodiment Construction
[0055] The present invention will be further described below in conjunction with accompanying drawing:
[0056] The crowd counting method based on deep learning target detection of the present invention comprises the following steps:
[0057] Step 1: Build a deep learning network model: use the YOLO3 network based on the existing DarkNet network; such as figure 1 As shown, in this step, the YOLO3 network is based on the basic network of the DarkNet network, and three scale extraction features are added, namely Scale1, Scale2, and Scale3. Among them, Scale1 adds some convolutional layers after the basic network and then outputs the box Information; Scale2 is upsampled from the convolutional layer of the penultimate layer in Scale1, and then added to the last 16x16 feature map, and then output box information after multiple convolutions. The scale is twice as large as Scale1: Scale3 Similar to Scale2, the final output feature map is of size 32×32.
[0058] Compared with other ...
PUM
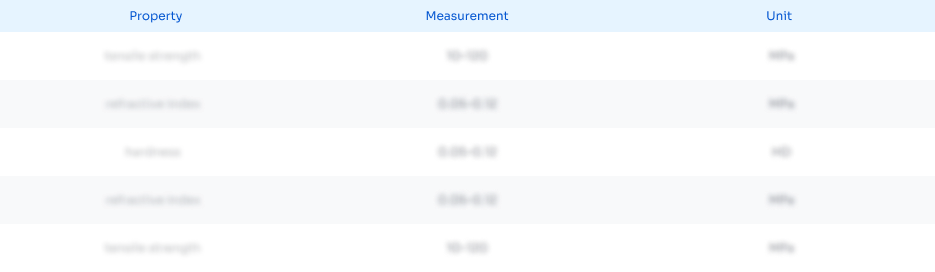
Abstract
Description
Claims
Application Information
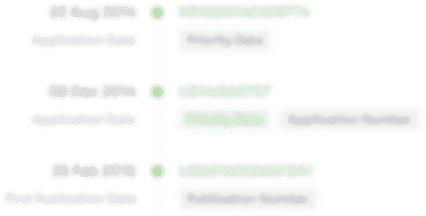
- R&D Engineer
- R&D Manager
- IP Professional
- Industry Leading Data Capabilities
- Powerful AI technology
- Patent DNA Extraction
Browse by: Latest US Patents, China's latest patents, Technical Efficacy Thesaurus, Application Domain, Technology Topic, Popular Technical Reports.
© 2024 PatSnap. All rights reserved.Legal|Privacy policy|Modern Slavery Act Transparency Statement|Sitemap|About US| Contact US: help@patsnap.com