Protein secondary structure prediction method based on deep neural networks
A deep neural network and secondary structure technology, applied in the field of protein secondary structure prediction based on deep neural network, can solve the problems of low accuracy, unable to meet daily application requirements, and few methods for predicting 8 states of proteins, etc. The effect of improving feature extraction capabilities
- Summary
- Abstract
- Description
- Claims
- Application Information
AI Technical Summary
Problems solved by technology
Method used
Image
Examples
Embodiment Construction
[0019] The technical solution of the present invention will be further described below in conjunction with specific embodiments.
[0020] Such as figure 1 As shown, the specific implementation of the present invention is to provide a protein secondary structure prediction method based on deep neural network, including the following steps:
[0021] Step 100: Extract protein sequence features. Among the input features of the network, the number of features of amino acid sequence information is 21, and the number of features of amino acid structure information is also 21. Each amino acid has 42 features that are used to predict its corresponding secondary level structure. Each protein sequence contains 700 amino acids at most, so each protein can be represented by a 700×42 matrix. If there are less than 700 amino acids in a protein, 0 is used to fill in the features of the following amino acid sequence. A protein sequence can be expressed as:
[0022]
[0023] Where L is the length of...
PUM
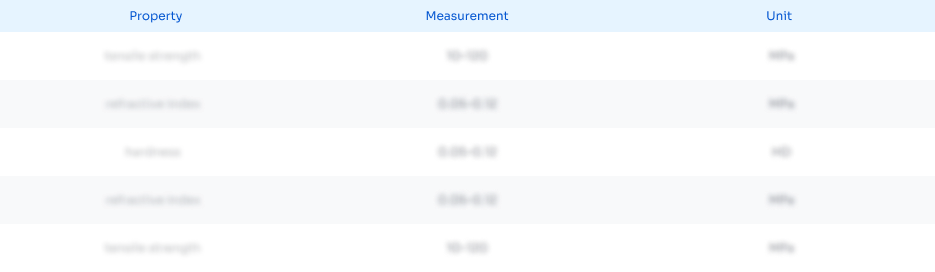
Abstract
Description
Claims
Application Information
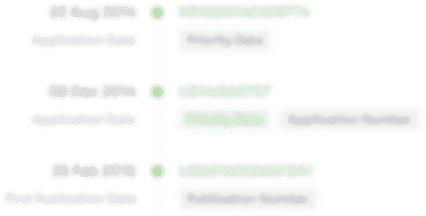
- R&D Engineer
- R&D Manager
- IP Professional
- Industry Leading Data Capabilities
- Powerful AI technology
- Patent DNA Extraction
Browse by: Latest US Patents, China's latest patents, Technical Efficacy Thesaurus, Application Domain, Technology Topic, Popular Technical Reports.
© 2024 PatSnap. All rights reserved.Legal|Privacy policy|Modern Slavery Act Transparency Statement|Sitemap|About US| Contact US: help@patsnap.com