A Triplet network learning method based on a semantic hierarchical structure
A technology of hierarchical structure and network learning, applied in machine learning, image enhancement, instruments, etc., can solve the problems of large network influence, slow convergence of network loss function, and no contribution to triplets in training, and achieves improved performance, enhanced image details, The effect of enhancing separability
- Summary
- Abstract
- Description
- Claims
- Application Information
AI Technical Summary
Problems solved by technology
Method used
Image
Examples
Embodiment Construction
[0043] The present invention will be further described below in conjunction with the accompanying drawings and embodiments.
[0044] The invention improves the performance of the Triplet network from a new research direction. Since the similarity between fine-grained sample categories is extremely high, the present invention considers integrating more abstract category information in the semantic level to improve the separability of fine-grained categories. The semantic hierarchy is a concept structure proposed by Quillian and Collins in 1969. In the network structure, various concepts are organized together according to the logical relationship between the upper and lower levels. The higher the level of the concept, the higher the level of abstraction and generalization. Since the traditional N-way softmax ignores the inter-class correlation, and the semantic hierarchy can be used to sort out the intra-class similarity and inter-class difference that exist widely in large-sca...
PUM
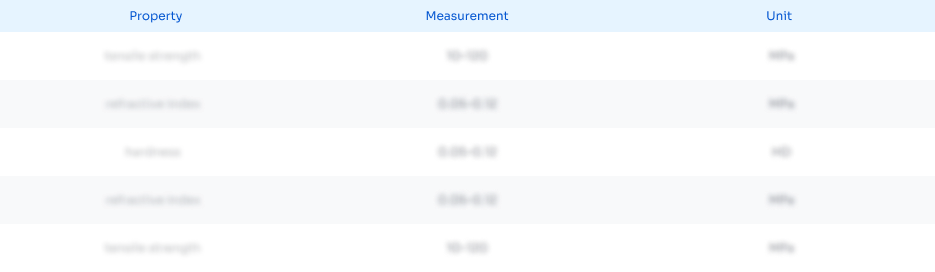
Abstract
Description
Claims
Application Information
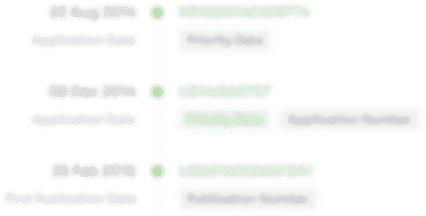
- R&D Engineer
- R&D Manager
- IP Professional
- Industry Leading Data Capabilities
- Powerful AI technology
- Patent DNA Extraction
Browse by: Latest US Patents, China's latest patents, Technical Efficacy Thesaurus, Application Domain, Technology Topic.
© 2024 PatSnap. All rights reserved.Legal|Privacy policy|Modern Slavery Act Transparency Statement|Sitemap