Mechanical vibration signal feature extraction method based on combination of stochastic resonance and kernel principal component analysis
A nuclear principal component analysis and stochastic resonance technology, applied in special data processing applications, instruments, electrical digital data processing, etc., can solve problems such as weak and nonlinear fault information and strong linear correlation that cannot be effectively reflected
- Summary
- Abstract
- Description
- Claims
- Application Information
AI Technical Summary
Problems solved by technology
Method used
Image
Examples
example
[0086] This example mainly verifies that the vibration feature extraction method based on the combination of stochastic resonance and nuclear principal component analysis can improve feature separability, eliminate linear correlation between features, and reduce feature dimension, thereby improving fault diagnosis accuracy and efficiency. Aeroengine rotor failure simulation test bench such as figure 1 As shown, its basic composition includes: a base 5, a first motor 3, a second motor 4, a first bearing 1, a second bearing 2, a coupling, a wheel, and the like. figure 1 is the structural schematic diagram of the test bench, where, B i (i=1,...,7) is the bearing seat, D i (i=1,…,4) is the rotor disk, P 1 ,P 2 ,P 3 for 3 vibration sensors, P 4 is the speed sensor, J 1 、J 2 、J 3 for the coupling.
[0087] The rotational speed of the first motor 3 is set at 1500rpm, and the rotational speed of the second motor 4 is set at 2400rpm, which is used to simulate the state where t...
PUM
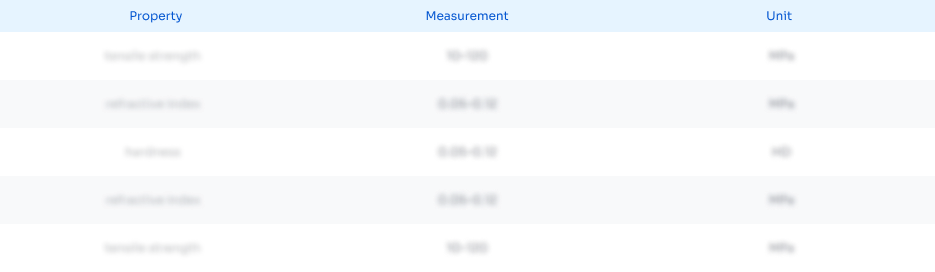
Abstract
Description
Claims
Application Information
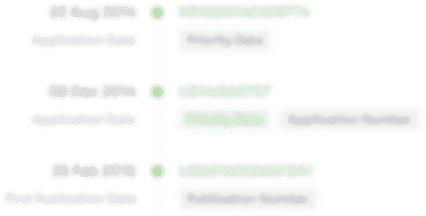
- R&D Engineer
- R&D Manager
- IP Professional
- Industry Leading Data Capabilities
- Powerful AI technology
- Patent DNA Extraction
Browse by: Latest US Patents, China's latest patents, Technical Efficacy Thesaurus, Application Domain, Technology Topic.
© 2024 PatSnap. All rights reserved.Legal|Privacy policy|Modern Slavery Act Transparency Statement|Sitemap