A deep deterministic strategy gradient learning method based on a reviewer and double experience pools
A Critic, Deterministic Technique for Deep Deterministic Policy Gradient Reinforcement Learning
- Summary
- Abstract
- Description
- Claims
- Application Information
AI Technical Summary
Problems solved by technology
Method used
Image
Examples
Embodiment Construction
[0088] In the following, specific embodiments of the present invention will be described in detail in conjunction with the examples and accompanying drawings. The embodiments depicted here are only used to illustrate and explain the present invention, but not to limit the present invention.
[0089] A deep deterministic policy gradient reinforcement learning method based on critics and double experience pools for intelligent unmanned systems proposed by the present invention mainly includes the following steps: first, analyze the environment where the agent is located and the actions of the agent and Determine the size of the observation space and action space of the agent. Based on this, the actor module and the critic module of the deep deterministic policy gradient method are constructed through the deep neural network, and the parameters are initialized randomly. Subsequently, multiple critics in the critic module are created. Each critic (critic) has a different structure...
PUM
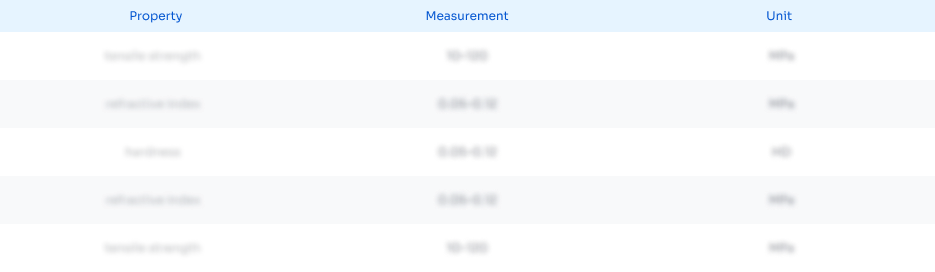
Abstract
Description
Claims
Application Information
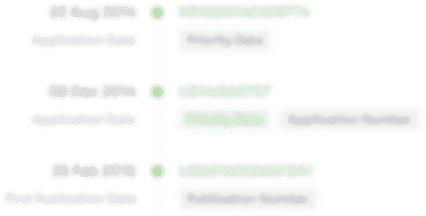
- R&D Engineer
- R&D Manager
- IP Professional
- Industry Leading Data Capabilities
- Powerful AI technology
- Patent DNA Extraction
Browse by: Latest US Patents, China's latest patents, Technical Efficacy Thesaurus, Application Domain, Technology Topic, Popular Technical Reports.
© 2024 PatSnap. All rights reserved.Legal|Privacy policy|Modern Slavery Act Transparency Statement|Sitemap|About US| Contact US: help@patsnap.com