Abnormal data detection method based on active learning
A technology of abnormal data detection and active learning, applied in the direction of integrated learning, etc., to achieve the effects of improving scalability, reducing workload, and high accuracy
- Summary
- Abstract
- Description
- Claims
- Application Information
AI Technical Summary
Problems solved by technology
Method used
Image
Examples
Embodiment Construction
[0032] The present invention is described in detail below with reference to accompanying drawing and embodiment:
[0033] attached Figure 1-4 It can be seen that an abnormal data detection method based on active learning,
[0034] According to the comparative analysis of various basic learners, 5 unsupervised models based on statistics-based Tukey Test and HBOS, similarity-based model iORCA, axis-parallel subspace division-based Isolation Forest and RSHash were selected as the base learners;
[0035] The outlier scores judged by each base learner are combined and presented to human experts for labeling;
[0036] This maximizes the amount of information that human experts can feed back.
[0037] A supervised binary classification model based on GBM (Gradient Boosting Machine) is trained by sampling 75% of the labeled data set and the data set generated by each basic learner vote, and the model is applied to the full data set to obtain the final mining results.
[0038] Fina...
PUM
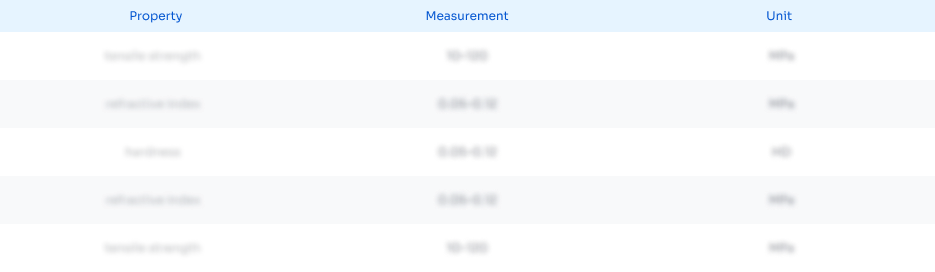
Abstract
Description
Claims
Application Information
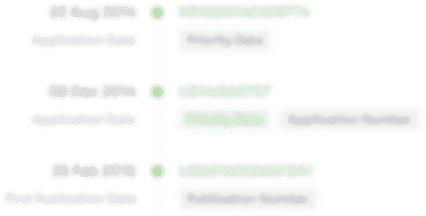
- R&D Engineer
- R&D Manager
- IP Professional
- Industry Leading Data Capabilities
- Powerful AI technology
- Patent DNA Extraction
Browse by: Latest US Patents, China's latest patents, Technical Efficacy Thesaurus, Application Domain, Technology Topic, Popular Technical Reports.
© 2024 PatSnap. All rights reserved.Legal|Privacy policy|Modern Slavery Act Transparency Statement|Sitemap|About US| Contact US: help@patsnap.com