Tibetan word vector representation method fusing components and character information
A word vector and component technology, applied in the field of Tibetan word vector representation that integrates components and word information, can solve the problems of few word vector learning objects, failure to obtain good word vectors, and insufficient semantic information mining, and achieve semantic and highly correlated effects
- Summary
- Abstract
- Description
- Claims
- Application Information
AI Technical Summary
Problems solved by technology
Method used
Image
Examples
specific Embodiment 1
[0100] For a given Tibetan word, it is decomposed into characters, and each character is further decomposed into several components. Tibetan words contain word meaning information, pre-added letters contain word tense and energy relationship information, super-added letters contain phonetic information and verb category information, base letters contain phonetic and tense information, and sub-added letters Letters contain phonological information, vowels contain morphological information, suffixes contain phonological, lexical, and grammatical information, and suffixed letters contain phonological, lexical, grammatical, and tense information, as well as degree information. For example, the Tibetan word (meaning students) can be decomposed into two words (meaning the present tense of learning) and (referring to the person suffix), word can be further broken down into components (base letter), (add letters below), (vowels) and (add letters after), these components...
specific Embodiment 2
[0101] Specific embodiment 2: The traditional CBOW word vector representation model represents " (World Progress)":
[0102] Such as Figure 5 As shown, the CBOW model learns the semantics of the target word from the "context" of the word in the corpus, and the word The semantics of the context word with acquired, i.e. word The word vector of the word consists of the word's context word with Obtained, vector splicing, summation or averaging operations are generally used when calculating word vectors;
specific Embodiment 3
[0103] Specific embodiment 3: TCCWEI model represents sentence " (World Progress)":
[0104] Such as Image 6 shown, word context words Character and word components co-acquisition. That is to say The word vector of and word and components The vectors of are obtained together.
PUM
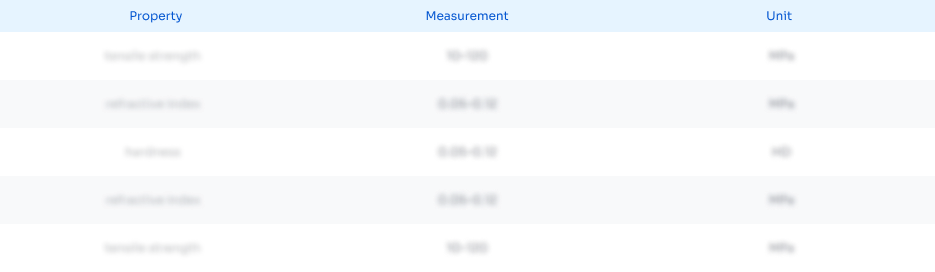
Abstract
Description
Claims
Application Information
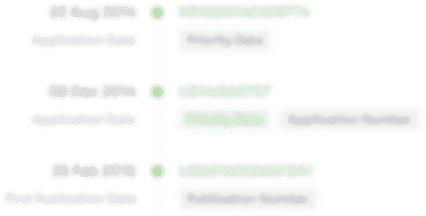
- R&D Engineer
- R&D Manager
- IP Professional
- Industry Leading Data Capabilities
- Powerful AI technology
- Patent DNA Extraction
Browse by: Latest US Patents, China's latest patents, Technical Efficacy Thesaurus, Application Domain, Technology Topic.
© 2024 PatSnap. All rights reserved.Legal|Privacy policy|Modern Slavery Act Transparency Statement|Sitemap