Short-term load prediction method based on incremental learning
A technology of short-term load forecasting and incremental learning, applied in forecasting, integrated learning, instruments, etc., can solve problems such as concept drift, forecasting model accuracy decline, and forecasting methods are no longer applicable, and achieve the effect of improving forecasting accuracy and realizing updates
- Summary
- Abstract
- Description
- Claims
- Application Information
AI Technical Summary
Problems solved by technology
Method used
Image
Examples
Embodiment
[0051] figure 1 It is a flowchart of the short-term load forecasting method based on incremental learning in the present invention.
[0052] In this example, if figure 1 As shown, a short-term load forecasting method based on incremental learning of the present invention comprises the following steps:
[0053] S1. Build initial dataset and incremental dataset
[0054] Divide the historical load data by season to obtain the historical load data of the four phases of spring, summer, autumn and winter. Since the distribution of spring load data and summer load data is quite different, we mark spring load data as initial data set and summer load data as incremental data. set, and the data of the remaining two stages are not analyzed;
[0055] S2. Use the correlation analysis algorithm to analyze the initial data set and the incremental data set respectively and extract the input load data
[0056] S2.1. Use the correlation analysis algorithm formula to calculate the correlation ...
PUM
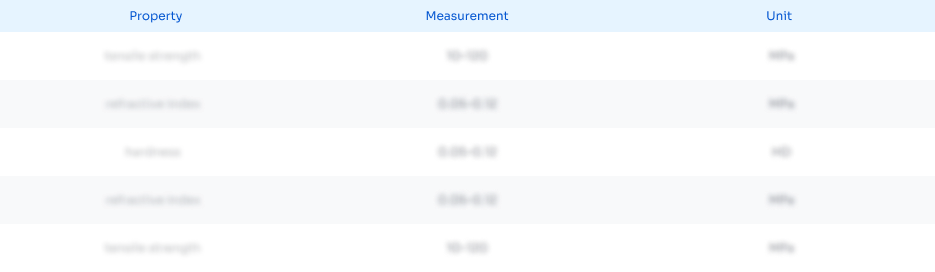
Abstract
Description
Claims
Application Information
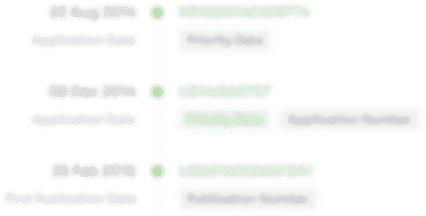
- R&D Engineer
- R&D Manager
- IP Professional
- Industry Leading Data Capabilities
- Powerful AI technology
- Patent DNA Extraction
Browse by: Latest US Patents, China's latest patents, Technical Efficacy Thesaurus, Application Domain, Technology Topic, Popular Technical Reports.
© 2024 PatSnap. All rights reserved.Legal|Privacy policy|Modern Slavery Act Transparency Statement|Sitemap|About US| Contact US: help@patsnap.com