Causal relationship mining method based on deep learning
A causal relationship, deep learning technology, applied in neural learning methods, neural architecture, biological neural network models, etc., can solve the problem that nonlinear data cannot be predicted with high accuracy
- Summary
- Abstract
- Description
- Claims
- Application Information
AI Technical Summary
Problems solved by technology
Method used
Image
Examples
Embodiment Construction
[0035] The present invention will be described in further detail below in conjunction with specific embodiments and with reference to the accompanying drawings.
[0036] The used hardware equipment of the present invention has 1 PC machine, 2 1080 graphics cards;
[0037] Such as image 3 As shown, the present invention provides a method for mining Granger causality based on deep learning, which specifically includes the following steps:
[0038] Step 1. Obtain relevant time series data and clean the data.
[0039] Step 2, preprocessing the data for data missing, not smooth, etc.
[0040] Step 2.1, for the missing values in the data, use the mode or median to complete.
[0041] In step 2.2, One-hot encoding or LabelEncoder is required to standardize category features.
[0042] Step 2.3, for the non-stationary time series, transform it into a stationary series through the method of first-order difference processing.
[0043] Step 2.4, convert the time series problem into...
PUM
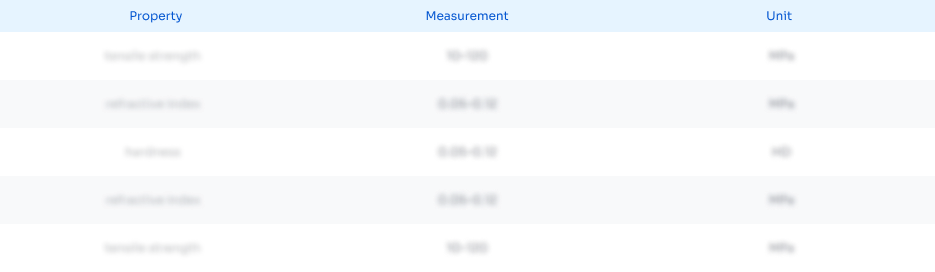
Abstract
Description
Claims
Application Information
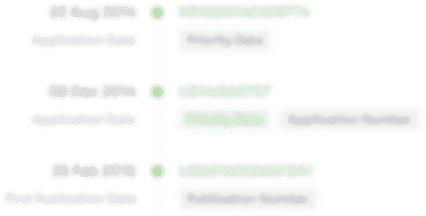
- R&D Engineer
- R&D Manager
- IP Professional
- Industry Leading Data Capabilities
- Powerful AI technology
- Patent DNA Extraction
Browse by: Latest US Patents, China's latest patents, Technical Efficacy Thesaurus, Application Domain, Technology Topic, Popular Technical Reports.
© 2024 PatSnap. All rights reserved.Legal|Privacy policy|Modern Slavery Act Transparency Statement|Sitemap|About US| Contact US: help@patsnap.com