Detection model compression method based on semantic segmentation
A semantic segmentation and detection model technology, applied in neural learning methods, biological neural network models, special data processing applications, etc., can solve the problems of not considering the correlation of parameters and low model compression ratio
- Summary
- Abstract
- Description
- Claims
- Application Information
AI Technical Summary
Problems solved by technology
Method used
Image
Examples
Embodiment Construction
[0033] see figure 1 , the present invention a kind of detection model compression method based on semantic segmentation, its steps are:
[0034] (1) Pruning:
[0035] 1) Input the convolution kernel weight.
[0036] 2) Prune the trained network model to remove redundant weights in the model that are less than a certain threshold. The method is to replace these weight values with 0, and obtain the parameter space of sparse weights through the pruning algorithm. And these 0 elements largely become auxiliary information for boundary search during semantic segmentation. can effectively help the semantic segmentation module.
[0037] (2) Semantic Segmentation:
[0038] 1) Perform semantic segmentation on the parameter space, obtain the hyperparameter block and the central vocabulary, and calculate the center position of the hyperparameter block, the method is:
[0039] Initially define the region of the parameter space, such as figure 2 shown.
[0040] Find the lowest...
PUM
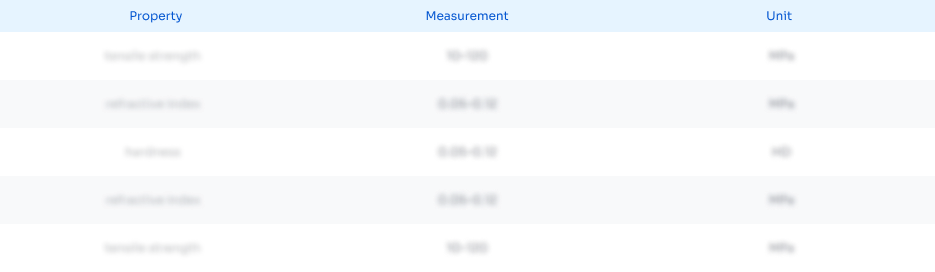
Abstract
Description
Claims
Application Information
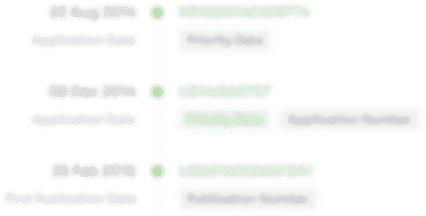
- Generate Ideas
- Intellectual Property
- Life Sciences
- Materials
- Tech Scout
- Unparalleled Data Quality
- Higher Quality Content
- 60% Fewer Hallucinations
Browse by: Latest US Patents, China's latest patents, Technical Efficacy Thesaurus, Application Domain, Technology Topic, Popular Technical Reports.
© 2025 PatSnap. All rights reserved.Legal|Privacy policy|Modern Slavery Act Transparency Statement|Sitemap|About US| Contact US: help@patsnap.com