User data reconstruction attack method oriented to deep federal learning
A user data and federation technology, which is applied in neural learning methods, digital data protection, electrical digital data processing, etc., can solve the problems of poor concealment, overpowering, privacy leakage, etc., and achieve the effect of improving concealment and authenticity
- Summary
- Abstract
- Description
- Claims
- Application Information
AI Technical Summary
Problems solved by technology
Method used
Image
Examples
Embodiment 1
[0074] 1) The malicious server participates in the regular federated learning process. First, the user agrees on the goal and model of collaborative learning, and then executes iteratively: the server sends the shared model, and the user trains the model locally and uploads the model parameters to the server. The terminal then aggregates these parameter updates until the model converges. The update of each round can be expressed as
[0075]
[0076] m t Indicates the shared model after round t update, Indicates that the t-th round of parameter update from user k is performed locally by private data pair M t calculated.
[0077] 2) The malicious server builds a multi-task generative adversarial network model locally, which includes a generative model G and a discriminative model D, where D simultaneously performs the task of discriminating the authenticity, category, and user identity of the input sample. The structure of the model can be expressed as
[0078] D. real...
PUM
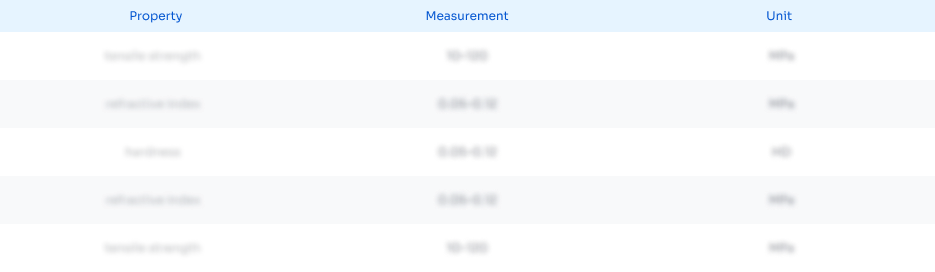
Abstract
Description
Claims
Application Information
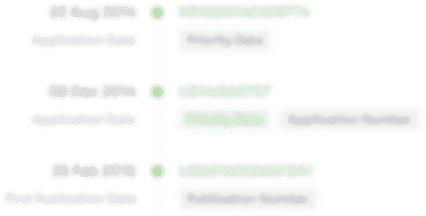
- R&D Engineer
- R&D Manager
- IP Professional
- Industry Leading Data Capabilities
- Powerful AI technology
- Patent DNA Extraction
Browse by: Latest US Patents, China's latest patents, Technical Efficacy Thesaurus, Application Domain, Technology Topic, Popular Technical Reports.
© 2024 PatSnap. All rights reserved.Legal|Privacy policy|Modern Slavery Act Transparency Statement|Sitemap|About US| Contact US: help@patsnap.com