A Transient Frequency Acquisition Method Based on Stacked Denoising Autoencoder
A noise reduction automatic encoding and transient frequency technology, applied in the electric power field, can solve problems such as difficult to comprehensively judge transient frequency stability, difficult to map input-output relationship, over-fitting or under-fitting problems, etc., to achieve good Pan-China capabilities and the effect of improving evaluation accuracy
- Summary
- Abstract
- Description
- Claims
- Application Information
AI Technical Summary
Problems solved by technology
Method used
Image
Examples
Embodiment Construction
[0097] The present invention will be further described below in conjunction with examples.
[0098] Such as figure 1 Shown is the dynamic change curve diagram of the system frequency under the condition of sudden load increase (ΔPL>0), and the present invention adopts the extreme value frequency f nadir , maximum rate of frequency change RoCoF, quasi-steady-state frequency f ss Measure frequency performance under active power disturbance events.
[0099] Therefore, the samples collected by the present invention include the extremum frequency f nadir , maximum rate of frequency change RoCoF, quasi-steady-state frequency f ss and power characteristic parameters. Among them, the power characteristic parameter is related to the extreme frequency f nadir , maximum rate of frequency change RoCoF, quasi-steady-state frequency f ss For closely related power parameters, the present invention selects a series of power parameters as feature categories, and then calculates the contr...
PUM
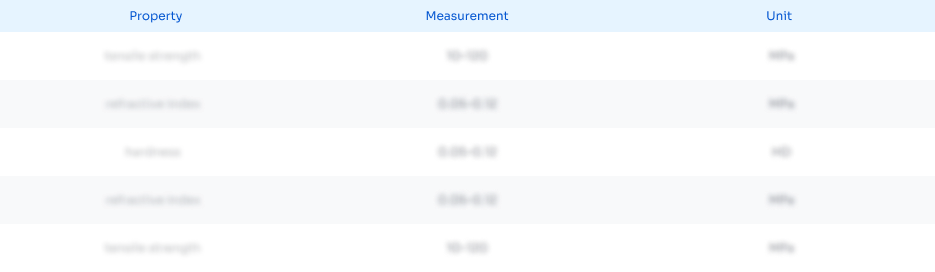
Abstract
Description
Claims
Application Information
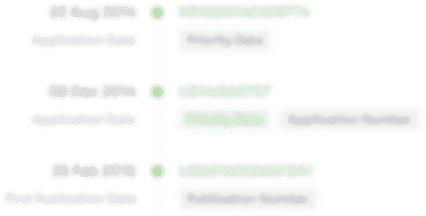
- R&D Engineer
- R&D Manager
- IP Professional
- Industry Leading Data Capabilities
- Powerful AI technology
- Patent DNA Extraction
Browse by: Latest US Patents, China's latest patents, Technical Efficacy Thesaurus, Application Domain, Technology Topic, Popular Technical Reports.
© 2024 PatSnap. All rights reserved.Legal|Privacy policy|Modern Slavery Act Transparency Statement|Sitemap|About US| Contact US: help@patsnap.com