Video action recognition method based on time perception structure
An action recognition and video technology, applied in the field of video recognition, can solve the problems of poor robustness, large amount of time modeling structure parameters, and high degree of dependence, and achieve the effect of improving the recognition rate, the recognition result, and the speed of overall convergence
- Summary
- Abstract
- Description
- Claims
- Application Information
AI Technical Summary
Problems solved by technology
Method used
Image
Examples
Embodiment 1
[0037] Such as figure 1 As shown, a video action recognition method based on time-aware structure, including the following steps:
[0038] A method for video action recognition based on a time-aware structure, comprising the following steps:
[0039] Step S1: Sparsely sample the original video data, extract n frames of the video at equal intervals, and use the video frames as the input frame data of the first two-dimensional convolutional neural network after data augmentation processing;
[0040] Step S2: Use the first two-dimensional convolutional neural network to process each input frame data of the original video separately to obtain deep features that are robust to changes in background, scale and illumination, and form a feature map t:
[0041] Step S3: Train the second two-dimensional convolutional neural network, use the trained second two-dimensional convolutional neural network as the monitoring flow network, and use the depth features output by a part of the convo...
Embodiment 2
[0058] Such as figure 1 , figure 2 As shown, in this embodiment, the UCF101 data set is taken as an example, and the size of each video frame in UCF101 is 240×320. Firstly, 5 frames of the video to be input are sparsely sampled, and then a 224×224 input image is cropped for each sampled frame using a random cropping method. In the present invention, the time-aware structure is embedded in figure 2 Between the i-d-3 layer and the i-e-1 layer.
[0059] (1) Two-dimensional convolution feature extraction of video frames
[0060] For each cropped input frame, use figure 2 i-a-1 to i-d-3 of the backbone network to extract the corresponding depth feature t i ∈ R 28×28×512 , in the process of processing different frames, the same set of convolutional network parameters is used, that is, the feature extraction process of different time periods shares parameters.
[0061] (2) Extraction of multi-scale spatio-temporal features
[0062] Two branches with time scales 3 and 5 are...
Embodiment 3
[0074] Such as image 3 , Figure 4 As shown, in this embodiment, in order to reflect the beneficial effect of the present invention, sufficient comparative experiments and elimination experiments have been done on the UCF101 data set.
[0075] There are a total of 13320 videos in UCF101, including human-computer interaction, human movement, playing musical instruments and other activities. UCF101 contains a large amount of background interference, variable shooting angles, large scale and illumination changes, and is a challenging dataset. In the process of testing, in order to be able to make a fair comparison with other methods, the first grouping method of UCF101 is used, that is, 9537 videos are used for training and 3783 videos are used for testing, and there is no overlap between them.
[0076] First, the time-aware structure and the effectiveness of the listener flow network are verified.
[0077] In order to demonstrate the advantages of the structure of the presen...
PUM
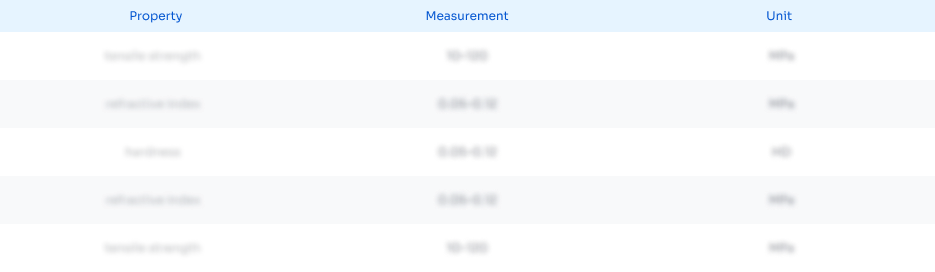
Abstract
Description
Claims
Application Information
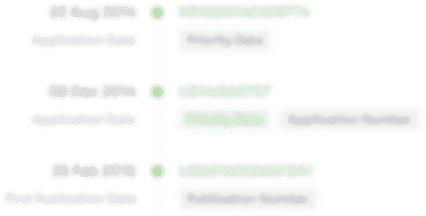
- R&D Engineer
- R&D Manager
- IP Professional
- Industry Leading Data Capabilities
- Powerful AI technology
- Patent DNA Extraction
Browse by: Latest US Patents, China's latest patents, Technical Efficacy Thesaurus, Application Domain, Technology Topic.
© 2024 PatSnap. All rights reserved.Legal|Privacy policy|Modern Slavery Act Transparency Statement|Sitemap