Cloud resource online scheduling method and device for distributed machine learning task
A machine learning and distributed technology, applied in the field of cloud computing, can solve problems such as poor scheduling effect
- Summary
- Abstract
- Description
- Claims
- Application Information
AI Technical Summary
Problems solved by technology
Method used
Image
Examples
Embodiment 1
[0094] This embodiment provides an online scheduling method for cloud resources oriented to distributed machine learning tasks, please refer to figure 1 , the method includes:
[0095] Step S1: At the beginning of each period, the cloud resource broker observes the price function of various resources in each geographically distributed data center and the amount of data that needs to be trained for each machine learning task. Among them, the geographically distributed data center uses For placing computing nodes and parameter servers, machine learning tasks are submitted by users, and at each moment, the amount of data that each task needs to be trained at the next moment is generated, and the price function is
[0096]
[0097] Among them, h is the resource usage, is the threshold, with For setting adjustable parameters based on actual resource prices.
[0098] Specifically, the inventors of the present application found through a lot of practice and research that ...
Embodiment 2
[0154] This embodiment provides an online scheduling device for cloud resources oriented to distributed machine learning tasks. Please refer to Figure 4 , the device consists of:
[0155] The price function and data volume observation module 201 is used to observe the price function of various resources in each geographically distributed data center and the amount of data that each machine learning task needs to train at the beginning of each period, wherein the geographical distribution The data center is used to place computing nodes and parameter servers. Machine learning tasks are submitted by users, and at each moment, the amount of data that each task needs to be trained at the next moment is generated. The price function is
[0156]
[0157] Among them, h is the resource usage, is the threshold, with To set adjustable parameters according to actual resource prices;
[0158] The cost calculation module 202 is used to calculate the cost generated in the proce...
Embodiment 3
[0185] See Figure 5 , based on the same inventive concept, the present application also provides a computer-readable storage medium 300, on which a computer program 311 is stored, and when the program is executed, the method as described in the first embodiment is implemented.
[0186] Since the computer-readable storage medium introduced in the third embodiment of the present invention is a computer device used to implement the cloud resource online scheduling method for distributed machine learning tasks in the first embodiment of the present invention, based on the introduction in the first embodiment of the present invention method, those skilled in the art can understand the specific structure and deformation of the computer-readable storage medium, so details are not repeated here. All computer-readable storage media used in the method in Embodiment 1 of the present invention fall within the scope of protection intended by the present invention.
PUM
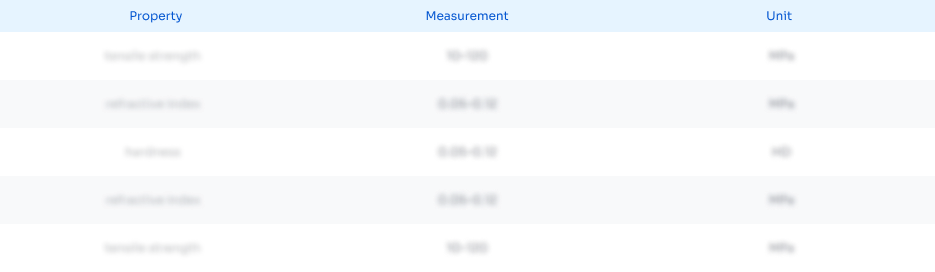
Abstract
Description
Claims
Application Information
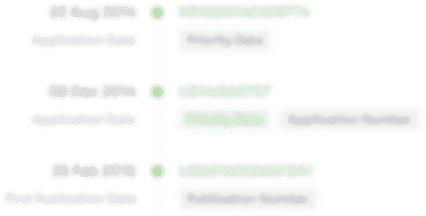
- R&D Engineer
- R&D Manager
- IP Professional
- Industry Leading Data Capabilities
- Powerful AI technology
- Patent DNA Extraction
Browse by: Latest US Patents, China's latest patents, Technical Efficacy Thesaurus, Application Domain, Technology Topic, Popular Technical Reports.
© 2024 PatSnap. All rights reserved.Legal|Privacy policy|Modern Slavery Act Transparency Statement|Sitemap|About US| Contact US: help@patsnap.com