Multi-label data flow classification method based on incremental learning
A classification method and incremental learning technology, applied in the field of multi-label classification, can solve problems such as not being able to detect and deal with concept drift in time
- Summary
- Abstract
- Description
- Claims
- Application Information
AI Technical Summary
Problems solved by technology
Method used
Image
Examples
Embodiment Construction
[0105] In order to better understand the technical content of the present invention, specific examples and accompanying drawings are described as follows.
[0106] figure 1 It is the overall flowchart of the multi-label data stream classification method based on the data block mechanism and incremental learning in the embodiment. As shown in the figure, the method mainly includes three stages, which are the initial training stage, the concept drift detection stage, and the ze
[0107] to combine figure 2 The implementation steps of the concept drift detection stage are described as follows:
[0108] Step 1. First, carry out concept drift detection at the data level. For two data blocks D 1 ,D 2 , to calculate the difference measure of the attribute;
[0109] Step 2 Calculate D 1 ,D 2 The label difference measure of ;
[0110] Step 3 Calculate D 1 ,D 2 The label density difference measure of ;
[0111] Step 4: Data block D 1 ,D 2 The overall difference metric is comp...
PUM
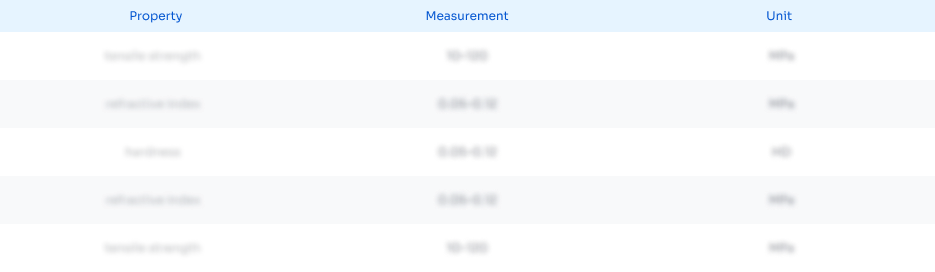
Abstract
Description
Claims
Application Information
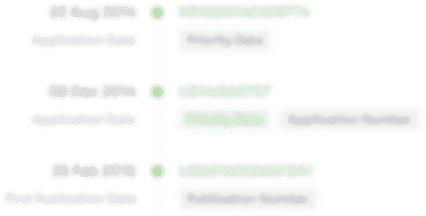
- R&D Engineer
- R&D Manager
- IP Professional
- Industry Leading Data Capabilities
- Powerful AI technology
- Patent DNA Extraction
Browse by: Latest US Patents, China's latest patents, Technical Efficacy Thesaurus, Application Domain, Technology Topic.
© 2024 PatSnap. All rights reserved.Legal|Privacy policy|Modern Slavery Act Transparency Statement|Sitemap