Personalized position recommendation method based on ensemble learning
An integrated learning and recommendation method technology, applied in integrated learning, special data processing applications, instruments, etc., can solve problems such as affecting the sales performance of e-commerce systems, reducing the quality of recommendation system services, and reducing the profits of service providers, so as to improve the use of Satisfaction, improving prediction accuracy, and improving user stickiness
- Summary
- Abstract
- Description
- Claims
- Application Information
AI Technical Summary
Problems solved by technology
Method used
Image
Examples
Embodiment Construction
[0040] The present invention will be described in further detail below in conjunction with the accompanying drawings and specific examples.
[0041] The specific process of the present invention's design and realization is as figure 2 The main variables and parameters in the process are shown in Table 1.
[0042] Table 1 Functions of main variables and parameters
[0043]
[0044]
[0045] The first step is to collect and organize the original user check-in data set C, and convert it into a user-location scoring matrix R. For the specific process, see image 3 , the operation steps are as follows:
[0046] (1.a) Select the user check-in data set C of the target recommender system. The data set consists of historical check-in records of U users to L addresses, and information such as user ID, address ID, access time, address longitude, and address latitude is extracted from each check-in record.
[0047] (1.b) Convert each check-in record into a triplet (u i , l j ...
PUM
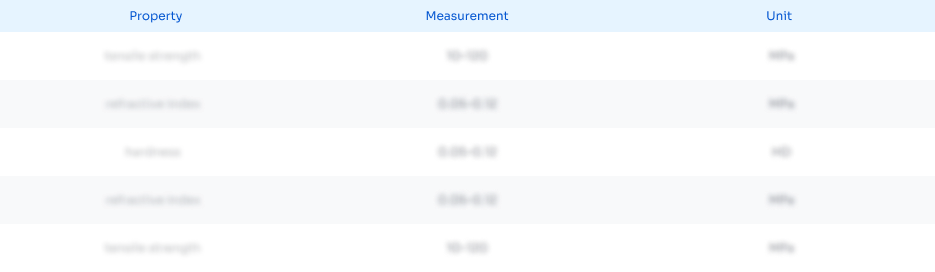
Abstract
Description
Claims
Application Information
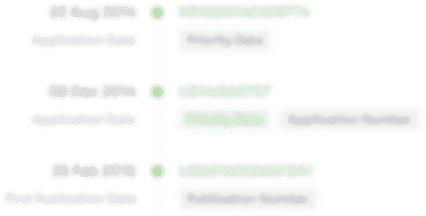
- R&D
- Intellectual Property
- Life Sciences
- Materials
- Tech Scout
- Unparalleled Data Quality
- Higher Quality Content
- 60% Fewer Hallucinations
Browse by: Latest US Patents, China's latest patents, Technical Efficacy Thesaurus, Application Domain, Technology Topic, Popular Technical Reports.
© 2025 PatSnap. All rights reserved.Legal|Privacy policy|Modern Slavery Act Transparency Statement|Sitemap|About US| Contact US: help@patsnap.com