Multi-target tracking method based on depth track prediction
A multi-target tracking and trajectory prediction technology, which is applied in the fields of computer vision and deep learning, can solve problems such as dynamic changes, uncertain number of targets, frequent occlusion between targets and interaction between targets with similar appearance, and achieve better robustness and improved effect of effect
- Summary
- Abstract
- Description
- Claims
- Application Information
AI Technical Summary
Problems solved by technology
Method used
Image
Examples
Embodiment Construction
[0037] In order to make the purpose, technical solution and advantages of the present invention clearer, the present invention will be further described in detail below in conjunction with the implementation methods and accompanying drawings.
[0038] see figure 1 , the specific implementation process of the multi-target tracking method based on depth trajectory prediction of the present invention, comprising the following steps:
[0039] Step 1. Build a depth trajectory prediction model:
[0040]Fully consider the historical trajectory information of the target and the scene information of the environment where the target is located, and construct an LSTM-based trajectory prediction model for the target in the multi-target tracking scene. The structure diagram of the trajectory prediction model is as follows: figure 2 shown.
[0041] Take m (m=1,...,insize) time as an example to illustrate the calculation process of the hidden layer state of the model at m time:
[0042] ...
PUM
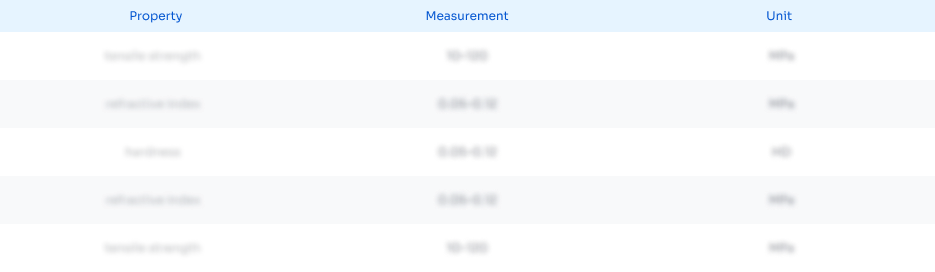
Abstract
Description
Claims
Application Information
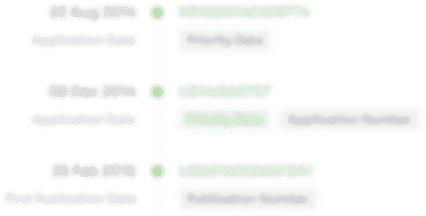
- Generate Ideas
- Intellectual Property
- Life Sciences
- Materials
- Tech Scout
- Unparalleled Data Quality
- Higher Quality Content
- 60% Fewer Hallucinations
Browse by: Latest US Patents, China's latest patents, Technical Efficacy Thesaurus, Application Domain, Technology Topic, Popular Technical Reports.
© 2025 PatSnap. All rights reserved.Legal|Privacy policy|Modern Slavery Act Transparency Statement|Sitemap|About US| Contact US: help@patsnap.com