3D point cloud data semantic segmentation method based on deep learning and self-attention
A technology of point cloud data and semantic segmentation, which is applied in image data processing, image analysis, character and pattern recognition, etc., can solve the problem of low segmentation accuracy and achieve the effect of improving accuracy
- Summary
- Abstract
- Description
- Claims
- Application Information
AI Technical Summary
Problems solved by technology
Method used
Image
Examples
Embodiment Construction
[0031] The present invention will be further described in detail below in conjunction with the accompanying drawings and specific embodiments.
[0032] refer to figure 1 , the present invention comprises the following steps:
[0033] Step 1) Get the training set R 2 and validation set V 2 :
[0034] Step 1a) Get the labeled 3D point cloud data file F from the database: {F 1 ,F 2 ,...,F i ,...,F f ,}, and the ratio is n R The 3D point cloud data file as the initial training set R 0 , the remaining f(1-n R ) 3D point cloud data files as the initial verification set V 0 , F i Indicates the i-th 3D point cloud data file, f is the total number of 3D point cloud data files, f≥100, 0.6≤n R R = 0.8, so that 80% of the 3D point cloud data files will be randomly selected from the database as the initial training set R 0 , and the remaining 20% of the 3D point cloud data files are used as the initial verification set V 0 ;
[0035] Step 1b) Add R 0 Input the PDAL library ...
PUM
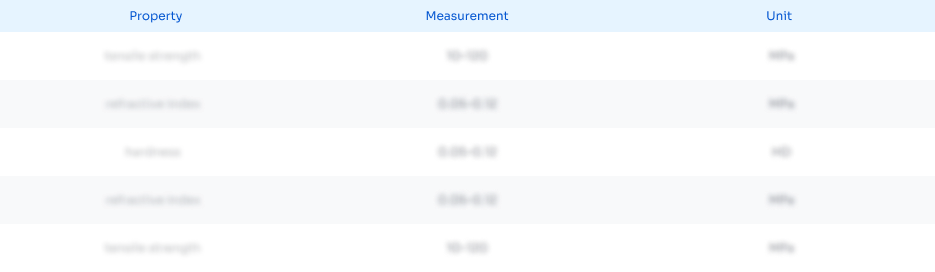
Abstract
Description
Claims
Application Information
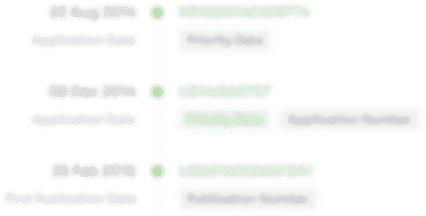
- R&D
- Intellectual Property
- Life Sciences
- Materials
- Tech Scout
- Unparalleled Data Quality
- Higher Quality Content
- 60% Fewer Hallucinations
Browse by: Latest US Patents, China's latest patents, Technical Efficacy Thesaurus, Application Domain, Technology Topic, Popular Technical Reports.
© 2025 PatSnap. All rights reserved.Legal|Privacy policy|Modern Slavery Act Transparency Statement|Sitemap|About US| Contact US: help@patsnap.com