Laser scanning SLAM indoor three-dimensional point cloud quality evaluation method based on deep learning
A technology of laser scanning and deep learning, applied in image data processing, instruments, character and pattern recognition, etc., can solve problems such as high incident angle, no frame and system, and SLAM system accuracy is not objective enough
- Summary
- Abstract
- Description
- Claims
- Application Information
AI Technical Summary
Problems solved by technology
Method used
Image
Examples
Embodiment
[0060] Please refer to figure 1 As shown, the present invention discloses a deep learning-based laser scanning SLAM indoor three-dimensional point cloud quality evaluation method, which mainly includes the following steps:
[0061] S1. Obtain high-quality point cloud and trajectory data through laser scanning SLAM device.
[0062] S2. Degrading the high-quality point cloud to obtain the simulation point cloud.
[0063] Specifically, step S2 specifically includes 3 steps:
[0064] S21. For the local characteristics of the point cloud trajectory, calculate whether the trajectory belongs to a straight line or a corner through curvature.
[0065] S22. Perform noise interference on the two tracks respectively. We first use standard Gaussian noise N(μ,σ^2) and a signal-to-noise ratio SNR within a certain threshold range to interfere with the correct track.
[0066] S23. Since the Gaussian noise is a random deviation, and the trajectory has a certain order and direction, the traje...
PUM
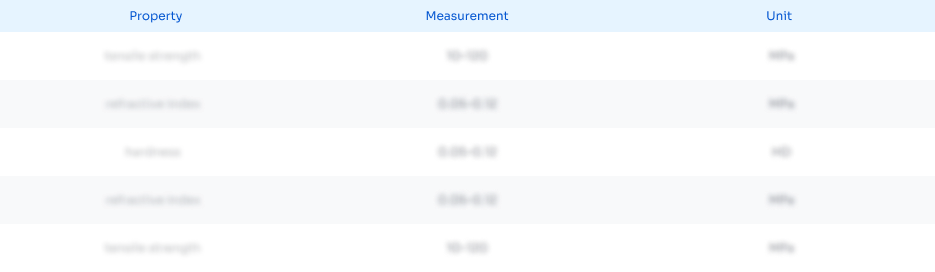
Abstract
Description
Claims
Application Information
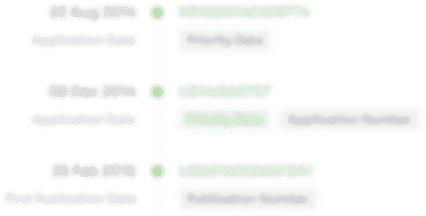
- R&D Engineer
- R&D Manager
- IP Professional
- Industry Leading Data Capabilities
- Powerful AI technology
- Patent DNA Extraction
Browse by: Latest US Patents, China's latest patents, Technical Efficacy Thesaurus, Application Domain, Technology Topic.
© 2024 PatSnap. All rights reserved.Legal|Privacy policy|Modern Slavery Act Transparency Statement|Sitemap