Enhanced learning method for calibrating beam deviation of accelerator
A technology of reinforcement learning and accelerators, applied in neural learning methods, biological neural network models, electrical components, etc., can solve problems such as high-energy safety hazards, track deviation, and inefficiency
- Summary
- Abstract
- Description
- Claims
- Application Information
AI Technical Summary
Problems solved by technology
Method used
Image
Examples
Embodiment Construction
[0018] In order to make the content, technical solutions and advantages of the present invention clearer, the present invention will be further described in detail in conjunction with the accompanying drawings. Modeling the environment for reinforcement learning in Figure-1:
[0019] Step 1): Establish a reinforcement learning method, mainly including environment, agent, calibration beam reward mechanism, deterministic strategy, etc.
[0020] Step 1.1: The environment is the calibration coil voltage value and position detector (BPM) value of the accelerator system, which can be directly read in the system (or directly read from the system database).
[0021] Step 1.2: The agent is the core component of deep reinforcement learning, and its specific neural network is described in step 4.2.
[0022] Step 1.3: The calibration beam reward mechanism is a reward and punishment rule for judging whether the position is good or bad according to the position after the beam position is c...
PUM
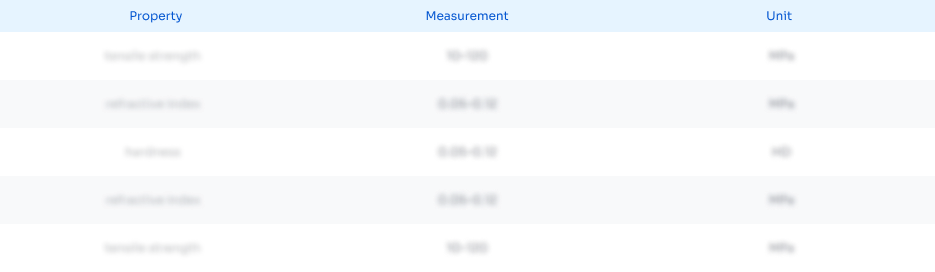
Abstract
Description
Claims
Application Information
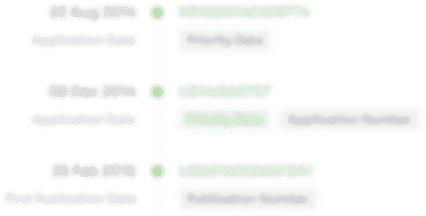
- Generate Ideas
- Intellectual Property
- Life Sciences
- Materials
- Tech Scout
- Unparalleled Data Quality
- Higher Quality Content
- 60% Fewer Hallucinations
Browse by: Latest US Patents, China's latest patents, Technical Efficacy Thesaurus, Application Domain, Technology Topic, Popular Technical Reports.
© 2025 PatSnap. All rights reserved.Legal|Privacy policy|Modern Slavery Act Transparency Statement|Sitemap|About US| Contact US: help@patsnap.com