Medical image noise reduction method based on generative adversarial network and 3D residual encoding and decoding
A technology of encoding and decoding and medical images, which is applied in the field of positron emission tomography image processing, can solve the problems of easy loss of image details and slow imaging speed, etc.
- Summary
- Abstract
- Description
- Claims
- Application Information
AI Technical Summary
Problems solved by technology
Method used
Image
Examples
Embodiment 1
[0045] Such as figure 2 As shown, a medical image denoising method based on generative adversarial network and 3D residual coding and decoding, image denoising for positron emission tomography, including: preprocessing the collected training data; using the processed data to train Convolutional neural network based on generative confrontation network and 3D residual encoding and decoding; use the trained convolutional neural network to denoise high-noise images to obtain high-quality images.
[0046] Data preprocessing includes:
[0047] Step A: The training data is provided by Neusoft Medical, such as Figure 3a As shown, it includes a low-quality whole-body scan image with a scan time of 75s and a high-quality whole-body scan image with a scan time of 150s, and the data format is DICOM, such as Figure 3b-3d As shown, these data can be roughly divided into three categories: head, lung and abdomen.
[0048]Step B: Convert the data in DICOM format into data in npy format w...
PUM
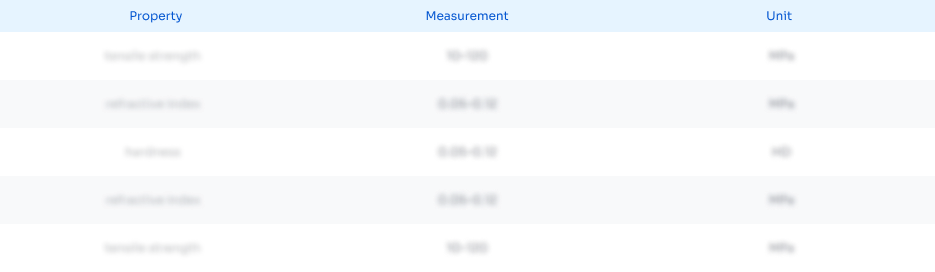
Abstract
Description
Claims
Application Information
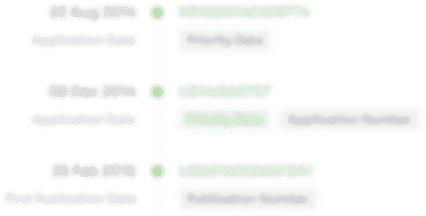
- R&D
- Intellectual Property
- Life Sciences
- Materials
- Tech Scout
- Unparalleled Data Quality
- Higher Quality Content
- 60% Fewer Hallucinations
Browse by: Latest US Patents, China's latest patents, Technical Efficacy Thesaurus, Application Domain, Technology Topic, Popular Technical Reports.
© 2025 PatSnap. All rights reserved.Legal|Privacy policy|Modern Slavery Act Transparency Statement|Sitemap|About US| Contact US: help@patsnap.com