Fine-grained image classification method and device based on spherical features
A classification method and fine-grained technology, applied in the direction of neural learning methods, instruments, biological neural network models, etc., can solve the problems of high computing time overhead, lack of visual understanding of images, differences, etc., to achieve small time complexity, efficient computing, The effect of reducing the number of total operations
- Summary
- Abstract
- Description
- Claims
- Application Information
AI Technical Summary
Problems solved by technology
Method used
Image
Examples
Embodiment 1
[0031] Such as figure 1 As shown, in terms of network construction, the technical solution of the present invention implements a fine-grained image classification method through three steps of construction.
[0032] (1) Extract general features of the input image using a general convolutional neural network
[0033] In the technical solution of the present invention, a general convolutional neural network will be used to extract common features of images. A general convolutional neural network is generally composed of multiple convolutional layer structures and pooling layer structures crossed in a specific order. Each convolution layer structure contains multiple convolution operations and activation operations for feature conversion and mapping. Each pooling layer structure includes a pooling operation for feature fusion. The output of the last convolutional layer structure is the general image feature X extracted by the entire general neural network. i .
[0034]The g...
Embodiment 2
[0056] Further, as Figure 5 As shown, it is a schematic diagram of the structure of the device for fine-grained image classification based on spherical features in the embodiment of the present invention. The device for fine-grained image classification based on spherical features in this embodiment includes one or more processors 21 and memory 22 . in, Figure 5 A processor 21 is taken as an example.
[0057] Processor 21 and memory 22 can be connected by bus or other means, Figure 5 Take connection via bus as an example.
[0058] Memory 22, as a non-volatile computer-readable storage medium, can be used to store non-volatile software programs and non-volatile computer-executable programs, such as the method for fine-grained image classification based on spherical features in Embodiment 1 . The processor 21 executes the method of fine-grained image classification based on spherical features by running non-volatile software programs and instructions stored in the memory...
PUM
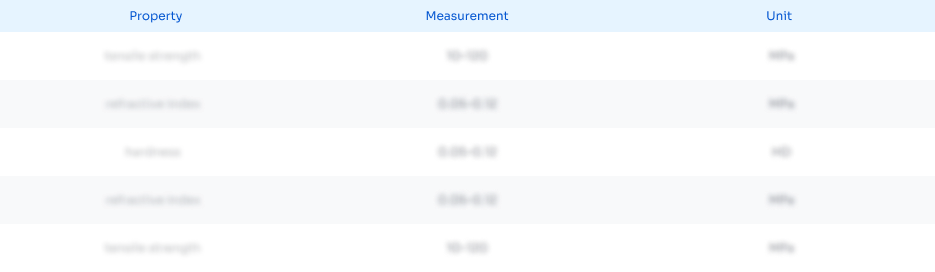
Abstract
Description
Claims
Application Information
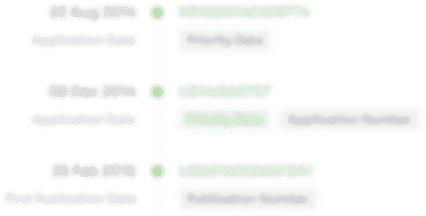
- R&D
- Intellectual Property
- Life Sciences
- Materials
- Tech Scout
- Unparalleled Data Quality
- Higher Quality Content
- 60% Fewer Hallucinations
Browse by: Latest US Patents, China's latest patents, Technical Efficacy Thesaurus, Application Domain, Technology Topic, Popular Technical Reports.
© 2025 PatSnap. All rights reserved.Legal|Privacy policy|Modern Slavery Act Transparency Statement|Sitemap|About US| Contact US: help@patsnap.com