SAR target image generation method with controllable azimuth angle
A target image and azimuth technology, applied in neural learning methods, biological neural network models, instruments, etc., can solve problems such as high dimensionality, inaccurate SAR image azimuth, and CGAN's inability to converge Nash equilibrium state, and achieve accurate azimuth. Effect
- Summary
- Abstract
- Description
- Claims
- Application Information
AI Technical Summary
Problems solved by technology
Method used
Image
Examples
example
[0126] In this example, image 3 and Figure 4 Generate the training result of the confrontational network for the condition in the present invention, wherein image 3 The change of the loss value of the discriminative model and the generative model during the training process of the CGAN model encoded for N-Progressive, Figure 4 SAR image generated for the N-Progressive coding based CGAN model. At the same time, the experimental results of CGAN based on two encoding methods, label encoding and one-hot encoding, are compared as follows: Figure 5 , Figure 6 , Figure 7 and Figure 8 shown.
[0127] According to the loss functions of the generative model and the discriminant model, after the model converges, the theoretical values of the generative model and the discriminant model are respectively:
[0128] G_loss=-log[D(fake)]=-log(0.5)=0.693
[0129] D_loss=-[log(D(real))+log(1-D(fake))]
[0130] =-[log(0.5)+log(1-0.5)]
[0131] =1.386
[0132] From Figure 5 ...
PUM
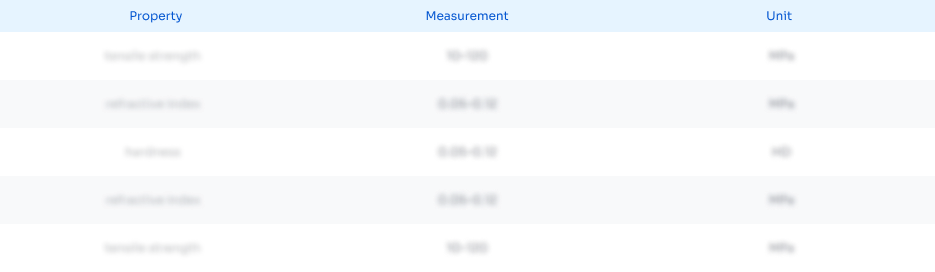
Abstract
Description
Claims
Application Information
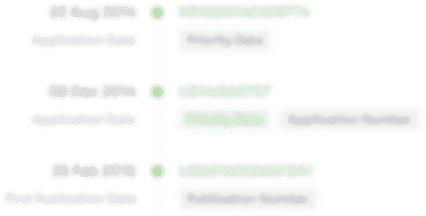
- R&D Engineer
- R&D Manager
- IP Professional
- Industry Leading Data Capabilities
- Powerful AI technology
- Patent DNA Extraction
Browse by: Latest US Patents, China's latest patents, Technical Efficacy Thesaurus, Application Domain, Technology Topic, Popular Technical Reports.
© 2024 PatSnap. All rights reserved.Legal|Privacy policy|Modern Slavery Act Transparency Statement|Sitemap|About US| Contact US: help@patsnap.com