Ship unloader association rule fault prediction model method based on deep belief network
A deep belief network and fault prediction technology, applied in biological neural network models, neural learning methods, loading/unloading, etc., can solve problems affecting machine functions, economic losses, accidents, etc., to enhance intelligence and solve high-dimensional problems Disaster and the effects of dealing with nonlinear data, removing complexity and ambiguity
- Summary
- Abstract
- Description
- Claims
- Application Information
AI Technical Summary
Problems solved by technology
Method used
Image
Examples
Embodiment Construction
[0058] The present invention will be further described below in conjunction with the accompanying drawings.
[0059] refer to Figure 1 to Figure 21 , a kind of ship unloader association rule fault prediction model method based on depth belief network, described prediction method comprises the following steps:
[0060] (1) The time series of the status monitoring of the trolley system of the bridge grab ship unloader is used as the model input, and the associated internal feature information and fault categories of the trolley system are obtained as the model output by sampling;
[0061] (2) Preprocess the original monitoring data, normalize the data, generate data sets and group them to obtain training sets and test sets;
[0062] (3) Reconstruct the phase space of the acquired time series T, convert it into a matrix form and construct the input and output y t ={x t}Map relationship f:R m → R;
[0063] (4) Train the deep belief network prediction model composed of RBM ...
PUM
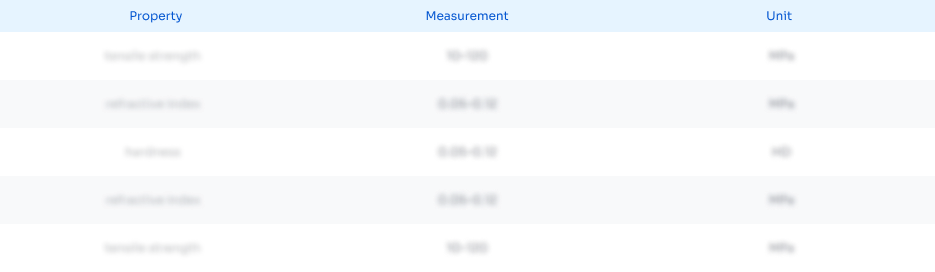
Abstract
Description
Claims
Application Information
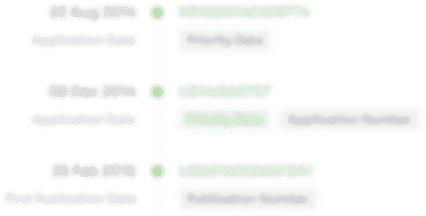
- R&D Engineer
- R&D Manager
- IP Professional
- Industry Leading Data Capabilities
- Powerful AI technology
- Patent DNA Extraction
Browse by: Latest US Patents, China's latest patents, Technical Efficacy Thesaurus, Application Domain, Technology Topic, Popular Technical Reports.
© 2024 PatSnap. All rights reserved.Legal|Privacy policy|Modern Slavery Act Transparency Statement|Sitemap|About US| Contact US: help@patsnap.com