Semantic segmentation method for low-illumination scene
A semantic segmentation and low-light technology, which is applied in the field of computer vision, can solve problems such as single action scenes, decreased accuracy, and lack of data sets, and achieve the effects of promoting convergence, accelerating convergence, and optimizing experimental results
- Summary
- Abstract
- Description
- Claims
- Application Information
AI Technical Summary
Problems solved by technology
Method used
Image
Examples
Embodiment Construction
[0035] (1) Network training
[0036] First import the parameters of the corresponding layers of the ResNet and DeepLabV3 networks to initialize a network to accelerate the training convergence after the network, that is, the pre-training of encoder C, encoder S and the final semantic segmentation part. Randomly group the collected data sets so that each group has an image of a low-light scene and an image of a normal scene, which are input to two encoders for corresponding feature extraction. This process is the retraining process after importing the ResNet pre-trained model. The encoder C part extracts the features in the low-light scene image and inputs them to the feature migration network part; the encoder S part extracts the features of the normal scene and passes through two multi-layer perceptrons (MLP), and the final features are combined with the encoder C Part of the output features are fused and migrated through the feature migration part. After the feature migrat...
PUM
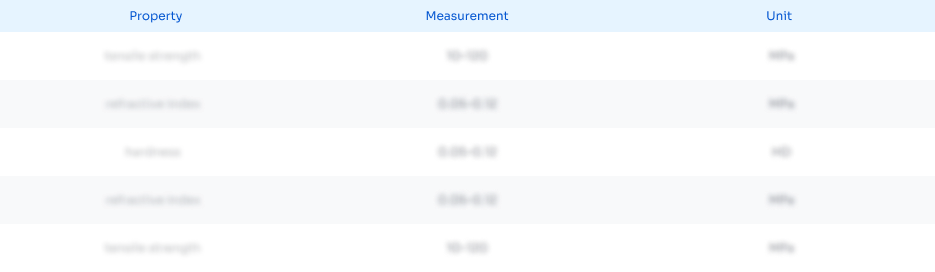
Abstract
Description
Claims
Application Information
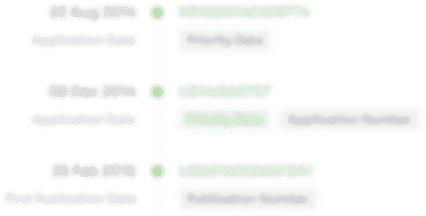
- R&D Engineer
- R&D Manager
- IP Professional
- Industry Leading Data Capabilities
- Powerful AI technology
- Patent DNA Extraction
Browse by: Latest US Patents, China's latest patents, Technical Efficacy Thesaurus, Application Domain, Technology Topic, Popular Technical Reports.
© 2024 PatSnap. All rights reserved.Legal|Privacy policy|Modern Slavery Act Transparency Statement|Sitemap|About US| Contact US: help@patsnap.com