Fabric defect pixel-level classification method based on deep learning
A defect pixel and deep learning technology, applied in the field of fabric defect pixel-level classification based on deep learning, can solve the problems of poor detection effect of complex textured fabrics, difficulty in meeting real-time performance, and poor accuracy, and achieve fast calculation speed and model The effect of small parameters and small amount of calculation
- Summary
- Abstract
- Description
- Claims
- Application Information
AI Technical Summary
Problems solved by technology
Method used
Image
Examples
Embodiment 1
[0074] Perform step 1, using the FID data set as the picture set;
[0075] Perform steps 2 to 5 to obtain a trained Mobile-Unet network model;
[0076] Perform step 6, select a picture from the test set as the input picture, the input picture is as follows Figure 7 shown; use the Mobile-Unet network model trained in step 5 to classify the input image, and output the classified image, such as Figure 8 shown.
Embodiment 2
[0078] Perform step 1, using the FID data set as the picture set;
[0079] Perform steps 2 to 5 to obtain a trained Mobile-Unet network model;
[0080] Perform step 6, select a picture from the test set as the input picture, the input picture is as follows Figure 9 shown; use the Mobile-Unet network model trained in step 5 to classify the input image, and output the classified image, such as Figure 10 shown.
Embodiment 3
[0082] Perform step 1, using the FID data set as the picture set;
[0083] Perform steps 2 to 5 to obtain a trained Mobile-Unet network model;
[0084] Perform step 6, select a picture from the test set as the input picture, the input picture is as follows Figure 11 shown; use the Mobile-Unet network model trained in step 5 to classify the input image, and output the classified image, such as Figure 12 shown.
PUM
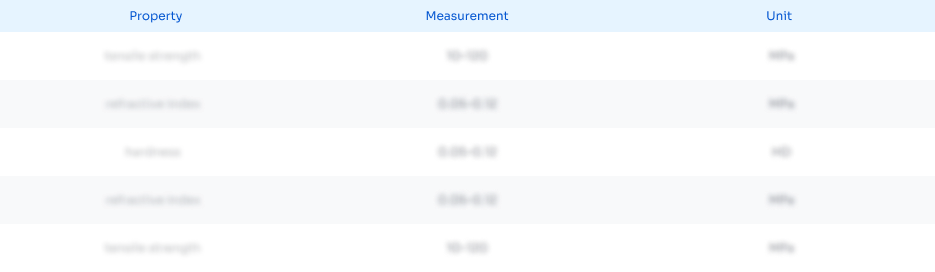
Abstract
Description
Claims
Application Information
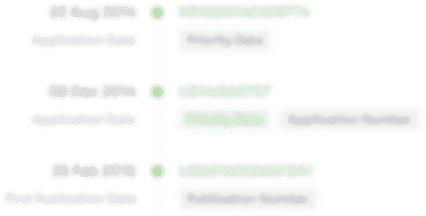
- R&D
- Intellectual Property
- Life Sciences
- Materials
- Tech Scout
- Unparalleled Data Quality
- Higher Quality Content
- 60% Fewer Hallucinations
Browse by: Latest US Patents, China's latest patents, Technical Efficacy Thesaurus, Application Domain, Technology Topic, Popular Technical Reports.
© 2025 PatSnap. All rights reserved.Legal|Privacy policy|Modern Slavery Act Transparency Statement|Sitemap|About US| Contact US: help@patsnap.com