Quantum-behaved particle swarm optimization (QPSO) recurrent predictor neural network (RPNN) method for financial time series prediction
A financial time series, recurrent neural network technology, applied in biological neural network models, finance, neural architecture, etc., can solve problems such as limiting long-term forecasting effects and unrealistic long-term forecasting, achieving less code writing workload and diversified expansion The effect of high stability and high prediction accuracy
- Summary
- Abstract
- Description
- Claims
- Application Information
AI Technical Summary
Problems solved by technology
Method used
Image
Examples
specific Embodiment
[0081] The closing price of the Shanghai Composite Index is the research object, and the data comes from the Wind Information Financial Terminal.
[0082] The data interval is from September 1, 2013 to November 1, 2016, with a total of 771 data.
[0083] The first 751 data (from September 1, 2013 to September 27, 2016) were used as training samples of the multi-branch time-delay recurrent neural network RPNN, and the network was trained with the QPSO optimization algorithm.
[0084] Such as image 3 As shown in , the trained RPNN is simulated (at this time, the RPNN has obtained the optimal network parameters, representing the nonlinear mapping F of the chaotic attractor in the reconstructed phase space), and the fitting situation between the simulated value and the sample is compared, and the test The generalization ability of the network.
[0085] Use the trained RPNN to make predictions. The last 20 data are used as prediction samples (September 28, 2016 to November 1, 2...
PUM
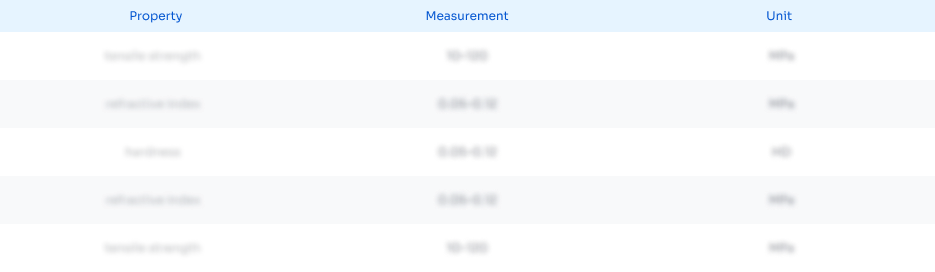
Abstract
Description
Claims
Application Information
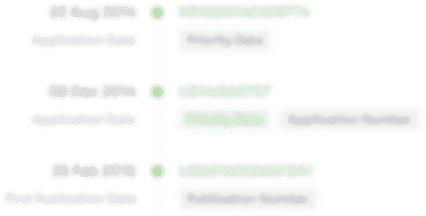
- R&D Engineer
- R&D Manager
- IP Professional
- Industry Leading Data Capabilities
- Powerful AI technology
- Patent DNA Extraction
Browse by: Latest US Patents, China's latest patents, Technical Efficacy Thesaurus, Application Domain, Technology Topic.
© 2024 PatSnap. All rights reserved.Legal|Privacy policy|Modern Slavery Act Transparency Statement|Sitemap