Target image tracking method based on deep reinforcement learning and space-time context
A spatiotemporal context, reinforcement learning technology, applied in the field of image processing, can solve the problems of tracking drift and slow tracking speed, and achieve the effect of avoiding tracking drift
- Summary
- Abstract
- Description
- Claims
- Application Information
AI Technical Summary
Problems solved by technology
Method used
Image
Examples
Embodiment Construction
[0052] In order to make the object, technical solution and advantages of the present invention more clear, the present invention will be further described in detail below in conjunction with the examples. It should be understood that the specific embodiments described here are only used to explain the present invention, not to limit the present invention.
[0053] 1) Model framework
[0054] Such as figure 2 As shown, at each time step t, the feature extraction network obtains an image x from the input sequence t . Visual features are generated by a feature extraction network. To obtain spatio-temporal features, visual features are first passed through STC and recurrent neural network. Then extract spatio-temporal features c from STC and RNN respectively t and the hidden state h t , where the spatiotemporal features c tWill be called ground-truth. In particular, the RNN also receives the previous hidden state h t-1 as input. In the final stage, at each time step t, ...
PUM
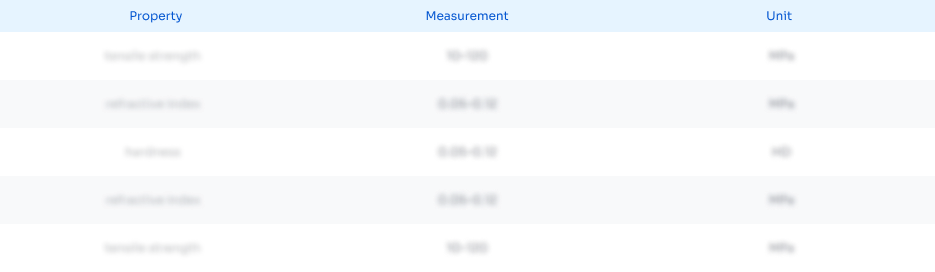
Abstract
Description
Claims
Application Information
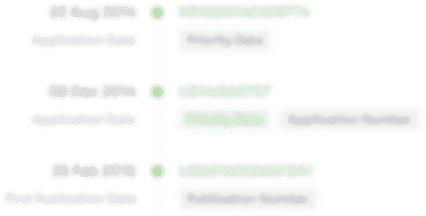
- R&D
- Intellectual Property
- Life Sciences
- Materials
- Tech Scout
- Unparalleled Data Quality
- Higher Quality Content
- 60% Fewer Hallucinations
Browse by: Latest US Patents, China's latest patents, Technical Efficacy Thesaurus, Application Domain, Technology Topic, Popular Technical Reports.
© 2025 PatSnap. All rights reserved.Legal|Privacy policy|Modern Slavery Act Transparency Statement|Sitemap|About US| Contact US: help@patsnap.com