Mechanical fault intelligent diagnosis method based on migration prototype network under small sample
A technology for mechanical failure and intelligent diagnosis, applied in neural learning methods, biological neural network models, computer components, etc., can solve problems such as the inability of intelligent diagnostic algorithms to learn fully, so as to improve generalization ability, improve learning, and get rid of dependence Effect
- Summary
- Abstract
- Description
- Claims
- Application Information
AI Technical Summary
Problems solved by technology
Method used
Image
Examples
Embodiment
[0053] The data set used contains four running states of the bearing. There are four running states of rolling bearing: normal, ball failure, inner ring failure and outer ring failure. Each running state contains 800 samples, which contains 3200 samples in total. Take 20 samples as training data, and the remaining 3180 samples as test data. The amount of training sample data only accounts for 0.625% of the total sample data. Take another data set containing the same four kinds of bearing operating states, where the data samples have different feature distributions, and take some of the samples as source domain data for auxiliary training.
[0054] Such as figure 1 As shown, the present invention includes the following steps:
[0055] Step 1: Perform standardized preprocessing on the obtained vibration signals of the four operating states of the rolling bearing, and use the zero-average normalization. The calculation formula is:
[0056]
[0057]
[0058]
[0059] Where n i Is the n...
PUM
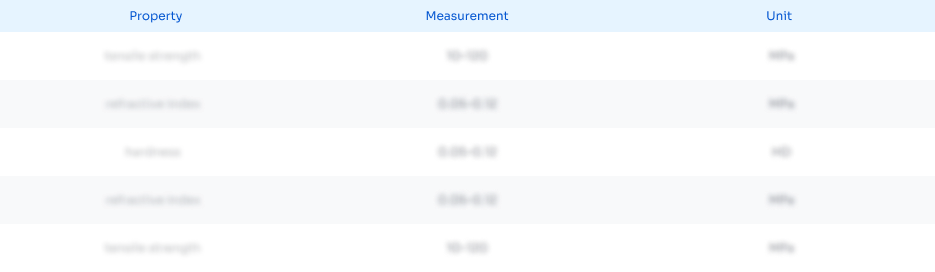
Abstract
Description
Claims
Application Information
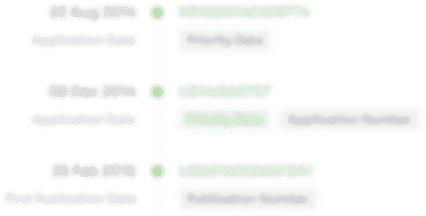
- R&D Engineer
- R&D Manager
- IP Professional
- Industry Leading Data Capabilities
- Powerful AI technology
- Patent DNA Extraction
Browse by: Latest US Patents, China's latest patents, Technical Efficacy Thesaurus, Application Domain, Technology Topic, Popular Technical Reports.
© 2024 PatSnap. All rights reserved.Legal|Privacy policy|Modern Slavery Act Transparency Statement|Sitemap|About US| Contact US: help@patsnap.com