Myoelectric gesture recognition method based on RNN-CNN architecture
A technology of gesture recognition and myoelectricity, which is applied in the field of physiological signal recognition, can solve problems such as improvement, long model training time, and affecting model accuracy
- Summary
- Abstract
- Description
- Claims
- Application Information
AI Technical Summary
Problems solved by technology
Method used
Image
Examples
Embodiment Construction
[0028] The technical solution of the present invention will be further described in detail below in conjunction with the accompanying drawings.
[0029]An EMG gesture recognition method based on the RNN-CNN architecture. According to the timing characteristics of the EMG signal, the gesture recognition method first uses the RNN architecture with a better processing sequence problem to perform feature extraction on each channel signal, and then uses the CNN architecture to perform feature extraction. The fused feature map is further extracted, which mainly includes the following steps:
[0030] Step 1: Data preprocessing.
[0031] Such as figure 1 As shown, after the EMG signal is obtained from the EMG acquisition device, the signal cannot generally be used directly, and several steps are required for signal processing. Assume that the sampling frequency of the EMG device is FHz, the sampling duration of each gesture is Tms, the number of channels of the device is C, and the...
PUM
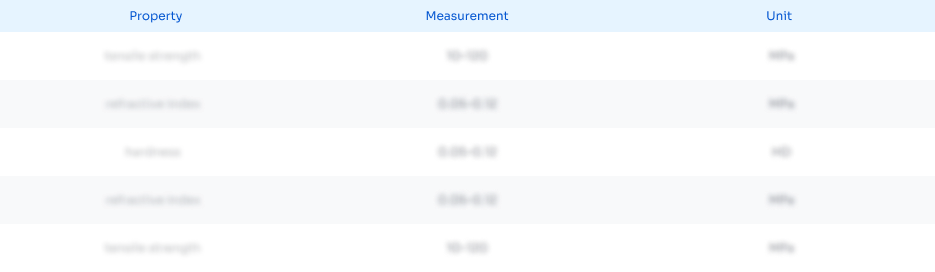
Abstract
Description
Claims
Application Information
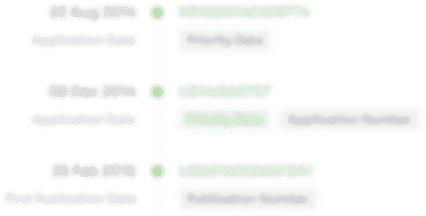
- R&D
- Intellectual Property
- Life Sciences
- Materials
- Tech Scout
- Unparalleled Data Quality
- Higher Quality Content
- 60% Fewer Hallucinations
Browse by: Latest US Patents, China's latest patents, Technical Efficacy Thesaurus, Application Domain, Technology Topic, Popular Technical Reports.
© 2025 PatSnap. All rights reserved.Legal|Privacy policy|Modern Slavery Act Transparency Statement|Sitemap|About US| Contact US: help@patsnap.com