Layered non-Gaussian process monitoring method based on common and specific feature extraction
A common feature and feature extraction technology, applied in character and pattern recognition, instruments, computer components, etc., can solve the problems of ignoring the interconnection of characteristics and commonness, and the poor effect of multi-modal process monitoring
- Summary
- Abstract
- Description
- Claims
- Application Information
AI Technical Summary
Problems solved by technology
Method used
Image
Examples
Embodiment Construction
[0089] The method of the present invention will be described in detail below in conjunction with the accompanying drawings and specific examples of implementation.
[0090] The specific implementation case adopted by the present invention is the Tennessee Eastman (TE) process, which includes five main units: reactor, condenser, compressor, separator and stripper.
[0091] The product stream from the reactor is cooled by a condenser before being sent to a vapor / liquid separator. The vapor from the separator is recycled to the reactor through the compressor. To prevent the build-up of inert components and reaction by-products in the process, a portion of the recycle stream must be vented. The condensed fraction (stream 10) from the separator is pumped to the stripper. Stream 4 is used to strip the remaining reactants in stream 10 which are combined via stream 5 with a recycle stream for the next reaction.
[0092] The Tennessee Eastman (TE) process has a total of 41 measured ...
PUM
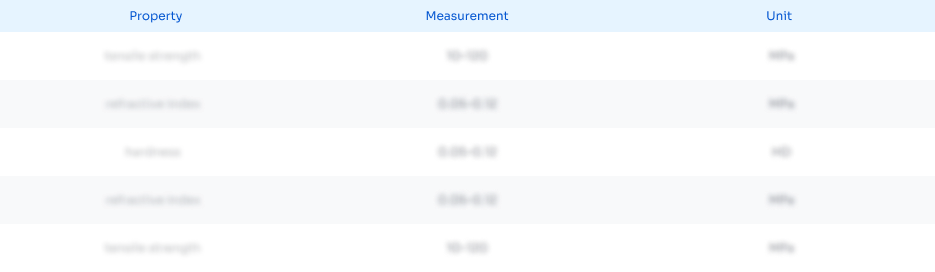
Abstract
Description
Claims
Application Information
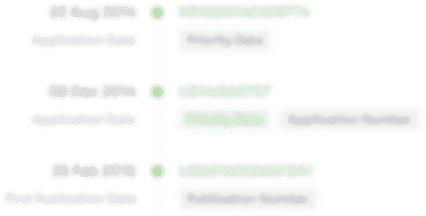
- R&D
- Intellectual Property
- Life Sciences
- Materials
- Tech Scout
- Unparalleled Data Quality
- Higher Quality Content
- 60% Fewer Hallucinations
Browse by: Latest US Patents, China's latest patents, Technical Efficacy Thesaurus, Application Domain, Technology Topic, Popular Technical Reports.
© 2025 PatSnap. All rights reserved.Legal|Privacy policy|Modern Slavery Act Transparency Statement|Sitemap|About US| Contact US: help@patsnap.com