Multi-scale difficulty vector classification method for graded reading materials
A classification method and multi-scale technology, applied in the direction of text database clustering/classification, special data processing applications, instruments, etc., can solve problems such as multi-time, limitation, insufficient sentence information extraction, etc., to enhance generalization and training. The effect of fast speed and rich difficulty feature representation
- Summary
- Abstract
- Description
- Claims
- Application Information
AI Technical Summary
Problems solved by technology
Method used
Image
Examples
Embodiment
[0032] figure 1 is a flowchart of the present invention, such as figure 1 As shown, a multi-scale difficulty vector classification method for graded readers disclosed in this embodiment includes the following steps: data cleaning, sentence segmentation, word segmentation, word-level feature extraction, sentence-level feature extraction, multi-scale feature extraction, splicing, GBDT Model training and feature importance analysis are as follows:
[0033] T1. Clean the original text data in the web html text format in advance, and then segment each sample into sentences. Chinese sentences can be segmented using the jieba tool, but not limited to this. Taking English data as an example here, such as figure 2 The sentence abbreviation "And it was... said" in the sentence and participle layer below puts the sentence " ‘And it was only10rubles for allthis,’ she said. ‘I’m taking it back for the girls at the factory to try.’ "After removing the html tag, it was divided into tw...
PUM
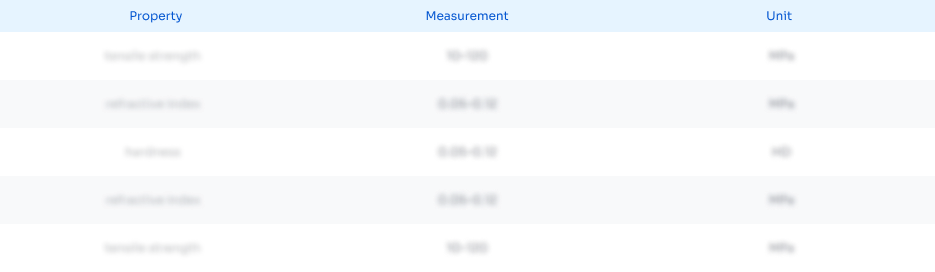
Abstract
Description
Claims
Application Information
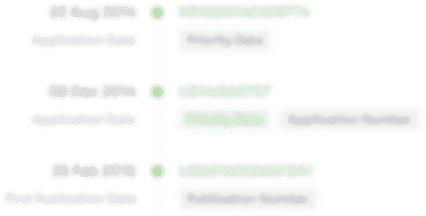
- R&D
- Intellectual Property
- Life Sciences
- Materials
- Tech Scout
- Unparalleled Data Quality
- Higher Quality Content
- 60% Fewer Hallucinations
Browse by: Latest US Patents, China's latest patents, Technical Efficacy Thesaurus, Application Domain, Technology Topic, Popular Technical Reports.
© 2025 PatSnap. All rights reserved.Legal|Privacy policy|Modern Slavery Act Transparency Statement|Sitemap|About US| Contact US: help@patsnap.com