A lightweight speech recognition method for edge computing
An edge computing and speech recognition technology, applied in the field of deep learning, can solve the problem of few applications of lightweight speech recognition network models, and achieve the effect of improving accuracy
- Summary
- Abstract
- Description
- Claims
- Application Information
AI Technical Summary
Problems solved by technology
Method used
Image
Examples
Embodiment
[0036] The lightweight speech recognition method for edge computing in this embodiment is characterized in that it includes the following steps:
[0037] S1. Construct a lightweight speech recognition network model EdgeRNN for edge computing devices, the EdgeRNN is composed of 1-D CNN and RNN, the 1-D CNN is used to extract spatial advanced features on temporal features, and the RNN For the modeling of speech time series; The EdgeRNN includes an acoustic feature extraction layer, a dense block, a maximum pooling layer, an RNN layer, a self-attention layer and a classification layer;
[0038] In speech recognition, it is first necessary to extract the acoustic features of the original speech. The present invention conducts multiple experiments from the two aspects of accuracy and speed, and finally selects and extracts 128-dimensional mel spectrogram, 12-dimensional delta and 12-dimensional double -delta feature.
[0039] This embodiment takes the four emotions in Session1 of ...
PUM
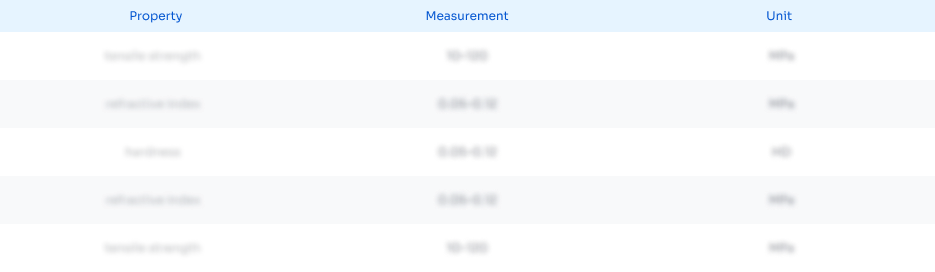
Abstract
Description
Claims
Application Information
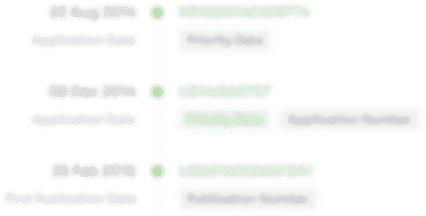
- R&D Engineer
- R&D Manager
- IP Professional
- Industry Leading Data Capabilities
- Powerful AI technology
- Patent DNA Extraction
Browse by: Latest US Patents, China's latest patents, Technical Efficacy Thesaurus, Application Domain, Technology Topic, Popular Technical Reports.
© 2024 PatSnap. All rights reserved.Legal|Privacy policy|Modern Slavery Act Transparency Statement|Sitemap|About US| Contact US: help@patsnap.com