End-to-end target guiding type conversation method based on deep learning
A goal-guided and deep learning technology, applied in the field of end-to-end goal-guided dialogue based on deep learning, can solve problems such as low dialogue efficiency and low accuracy, and achieve the effect of improving dialogue efficiency and enhancing personalized experience.
- Summary
- Abstract
- Description
- Claims
- Application Information
AI Technical Summary
Problems solved by technology
Method used
Image
Examples
Embodiment 1
[0031] see figure 1 , an end-to-end goal-guided dialogue method based on deep learning, including the following steps:
[0032] S1: Obtain the last round of user dialogue and the current dialogue history, and initialize the sequence-to-sequence model according to the previous round of user dialogue and the current dialogue history;
[0033] S2: Determine the line number of the current knowledge base entity;
[0034] S3: Determine the column number of the current knowledge base entity;
[0035] S4: Obtain the best matching entity through the attention mechanism;
[0036] S5: Steps S2-S4 are iteratively executed until the next round of dialogue is finally output.
[0037] The sequence-to-sequence model includes an encoder and a decoder. After the encoder is given the dialogue history and the user's last round of dialogue, the encoder encodes and abstracts the output content, obtains the last context vector, and transmits the context vector to the decoder. ; The decoder recei...
PUM
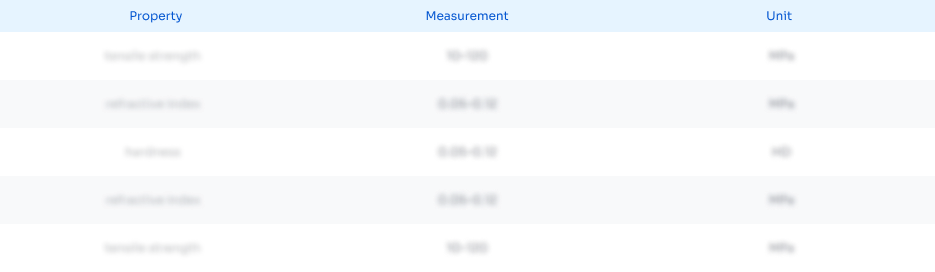
Abstract
Description
Claims
Application Information
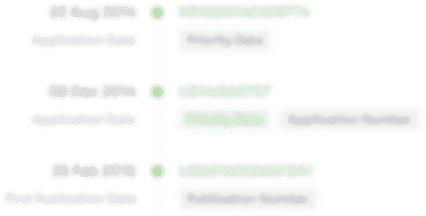
- R&D Engineer
- R&D Manager
- IP Professional
- Industry Leading Data Capabilities
- Powerful AI technology
- Patent DNA Extraction
Browse by: Latest US Patents, China's latest patents, Technical Efficacy Thesaurus, Application Domain, Technology Topic, Popular Technical Reports.
© 2024 PatSnap. All rights reserved.Legal|Privacy policy|Modern Slavery Act Transparency Statement|Sitemap|About US| Contact US: help@patsnap.com