A Fault Diagnosis Method for Deep Adversarial Transfer Networks Based on Wasserstein Distance
A fault diagnosis and distance technology, applied in the testing of mechanical components, testing of machine/structural components, instruments, etc., can solve problems such as unsatisfactory accuracy of classifiers
- Summary
- Abstract
- Description
- Claims
- Application Information
AI Technical Summary
Problems solved by technology
Method used
Image
Examples
Embodiment Construction
[0081] The following will clearly and completely describe the technical solutions in the embodiments of the present invention with reference to the accompanying drawings in the embodiments of the present invention. Obviously, the described embodiments are only some, not all, embodiments of the present invention. Based on the embodiments of the present invention, all other embodiments obtained by persons of ordinary skill in the art without making creative efforts belong to the protection scope of the present invention.
[0082] Depend on figure 1 As shown, a kind of fault diagnosis method based on the depth of Wasserstein distance against migration network of the present invention comprises the following steps:
[0083] S1, get the source domain D respectively s and target domain D t vibration data set. Among them, D means the field is the domain; the superscript s means the source, D s means the source domain; the superscript t means the target, D t i.e. the target domai...
PUM
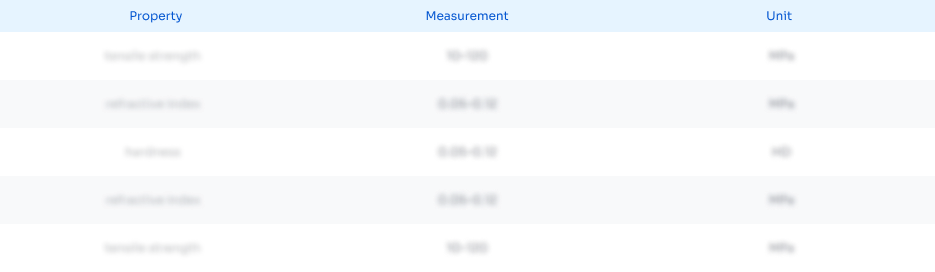
Abstract
Description
Claims
Application Information
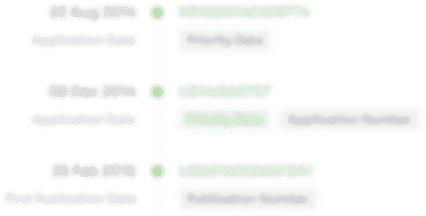
- R&D
- Intellectual Property
- Life Sciences
- Materials
- Tech Scout
- Unparalleled Data Quality
- Higher Quality Content
- 60% Fewer Hallucinations
Browse by: Latest US Patents, China's latest patents, Technical Efficacy Thesaurus, Application Domain, Technology Topic, Popular Technical Reports.
© 2025 PatSnap. All rights reserved.Legal|Privacy policy|Modern Slavery Act Transparency Statement|Sitemap|About US| Contact US: help@patsnap.com