A node failure prediction method for large-scale cluster systems
A fault prediction, cluster system technology, applied in information technology support systems, character and pattern recognition, biological neural network models, etc. The effect of high accuracy and strong adaptability
- Summary
- Abstract
- Description
- Claims
- Application Information
AI Technical Summary
Problems solved by technology
Method used
Image
Examples
Embodiment Construction
[0030] see Figure 4 , the present invention is a node failure prediction method for large-scale cluster systems. First, collect the resource occupancy data of each node, perform data processing to generate a data set, use a long short-term memory network (LSTM) to build a first data prediction model, and use a random forest. Build the second fault prediction model, establish the data of the first observation window, determine whether the size of the first observation window is equal to 3 hours, if not, return to rebuild; if it is satisfied, use the first data prediction model to predict the data in the advance time window, Combine the first observation window with the data in the advance time window to form a second observation window, and judge whether the size of the second observation window is equal to 4 hours, if not, return to rebuild the second observation window; if so, use the second fault prediction The model predicts failures within the prediction window.
[0031]...
PUM
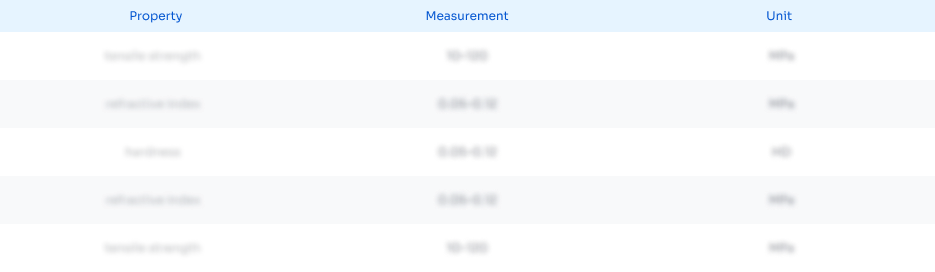
Abstract
Description
Claims
Application Information
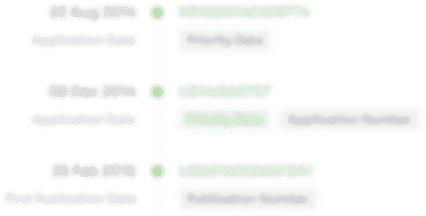
- R&D Engineer
- R&D Manager
- IP Professional
- Industry Leading Data Capabilities
- Powerful AI technology
- Patent DNA Extraction
Browse by: Latest US Patents, China's latest patents, Technical Efficacy Thesaurus, Application Domain, Technology Topic, Popular Technical Reports.
© 2024 PatSnap. All rights reserved.Legal|Privacy policy|Modern Slavery Act Transparency Statement|Sitemap|About US| Contact US: help@patsnap.com