Traffic characteristic prediction method and system and storage medium
A technology of traffic characteristics and prediction methods, applied in the field of intelligent transportation, can solve the problems of reducing the occupancy rate of video memory, low accuracy, and short training time.
- Summary
- Abstract
- Description
- Claims
- Application Information
AI Technical Summary
Problems solved by technology
Method used
Image
Examples
Embodiment 1
[0079] like figure 1 as shown, figure 1 It is a schematic diagram of the structure of the deep learning model GA-GCN.
[0080] The present invention describes some steps in the method in detail in conjunction with specific examples.
[0081] Step 1: Each road obtains an instant speed every 5 minutes, so each road has 288 instant speeds a day.
[0082] Step 2: Preprocess the data. If there are missing values in the input data, use the linear interpolation method to complete them, and divide the data into training set and test set in proportion. In the experimental data set, the California data uses 34 days as the training set, 5 days as the verification set, and 5 days as the test set; the Los Angeles data uses 5 days as the training set, 1 day as the verification set, and 1 day as the test set.
[0083] Step 3: Divide the data set, and the length of the historical time window is 60 minutes, that is, 12 known instant speeds are used to predict the speed of the next 15 minu...
PUM
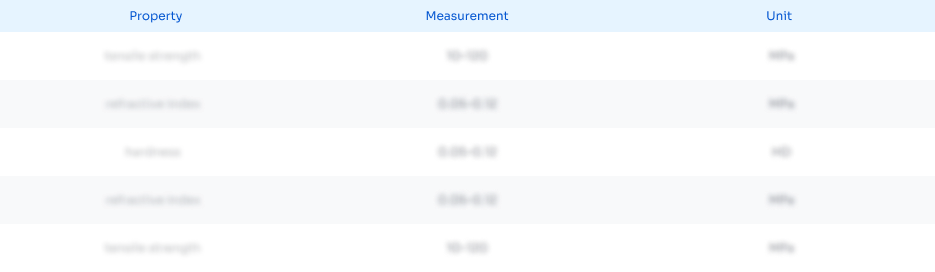
Abstract
Description
Claims
Application Information
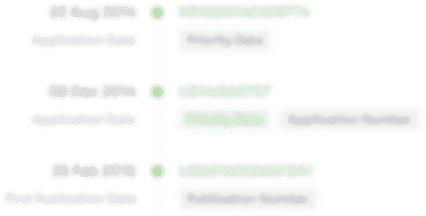
- Generate Ideas
- Intellectual Property
- Life Sciences
- Materials
- Tech Scout
- Unparalleled Data Quality
- Higher Quality Content
- 60% Fewer Hallucinations
Browse by: Latest US Patents, China's latest patents, Technical Efficacy Thesaurus, Application Domain, Technology Topic, Popular Technical Reports.
© 2025 PatSnap. All rights reserved.Legal|Privacy policy|Modern Slavery Act Transparency Statement|Sitemap|About US| Contact US: help@patsnap.com